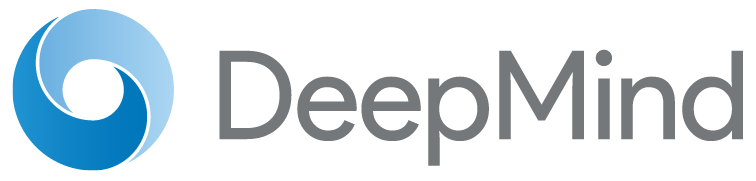
DeepMind
Founded Year
2010Stage
Acquired | AcquiredValuation
$0000About DeepMind
DeepMind specializes in advanced artificial intelligence research and development within the technology sector. The company's main offerings include creating artificial intelligence systems that aim to solve complex problems and contribute to scientific advancements. DeepMind's technologies are applied across various sectors, including healthcare for disease diagnosis, energy for data center efficiency, and science for protein structure prediction. It was founded in 2010 and is based in London, England. In January 2014, DeepMind was acquired by Google at a valuation between $500M and $650M.
Loading...
Loading...
Research containing DeepMind
Get data-driven expert analysis from the CB Insights Intelligence Unit.
CB Insights Intelligence Analysts have mentioned DeepMind in 7 CB Insights research briefs, most recently on Oct 7, 2024.

Jul 2, 2024 team_blog
How to buy AI: Assessing AI startups’ potential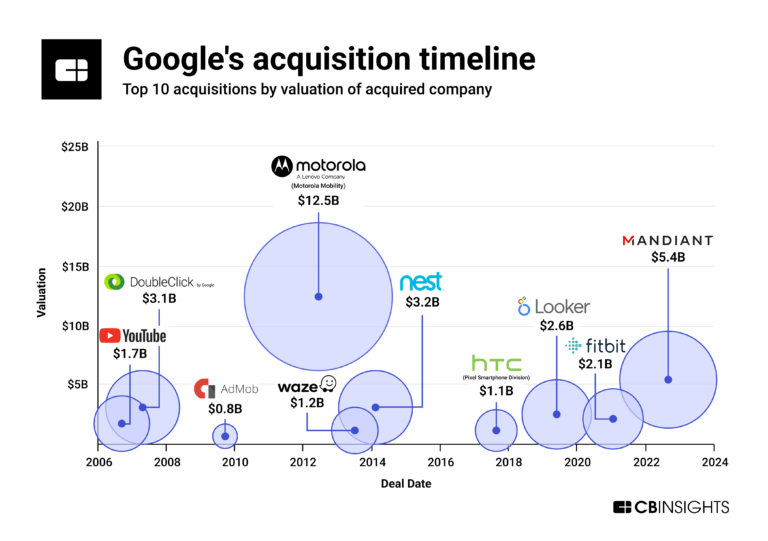
Apr 11, 2024
Google’s biggest acquisitions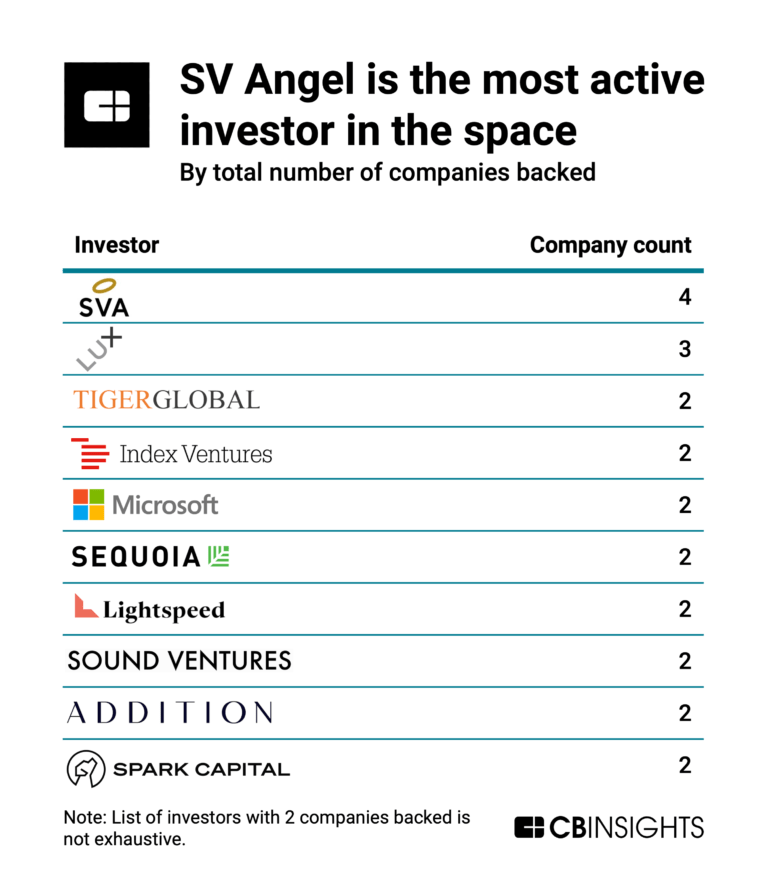
Jul 14, 2023
The state of LLM developers in 6 charts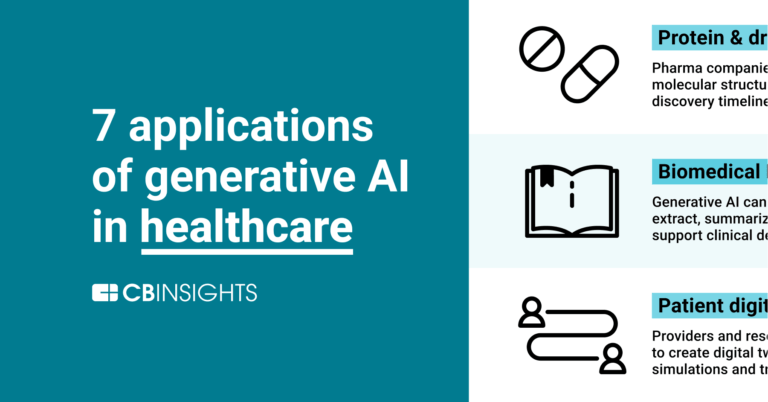
May 9, 2023
7 applications of generative AI in healthcare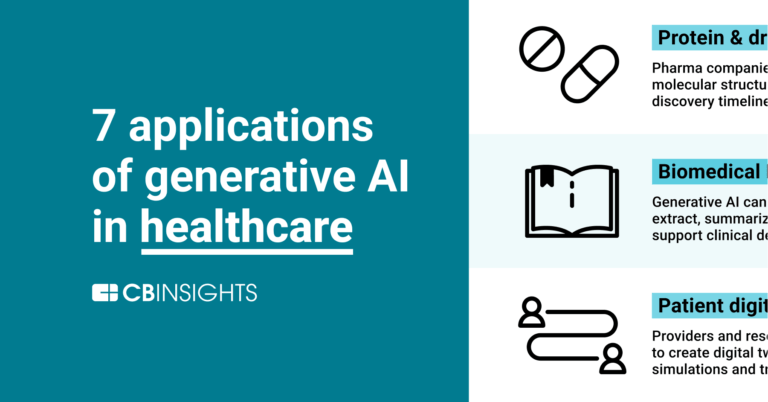
May 9, 2023
7 applications of generative AI in healthcareExpert Collections containing DeepMind
Expert Collections are analyst-curated lists that highlight the companies you need to know in the most important technology spaces.
DeepMind is included in 1 Expert Collection, including Artificial Intelligence.
Artificial Intelligence
9,378 items
Companies developing artificial intelligence solutions, including cross-industry applications, industry-specific products, and AI infrastructure solutions.
DeepMind Patents
DeepMind has filed 476 patents.
The 3 most popular patent topics include:
- artificial neural networks
- machine learning
- artificial intelligence
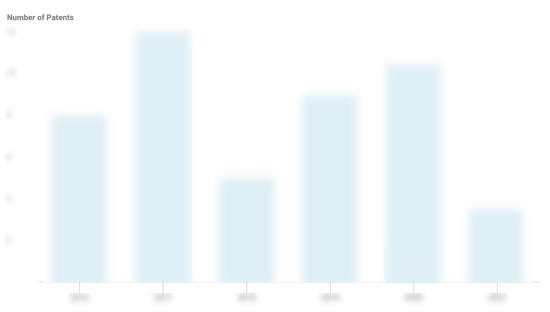
Application Date | Grant Date | Title | Related Topics | Status |
---|---|---|---|---|
4/6/2023 | 10/22/2024 | Artificial neural networks, Artificial intelligence, Rotating disc computer storage media, Classification algorithms, Computational neuroscience | Grant |
Application Date | 4/6/2023 |
---|---|
Grant Date | 10/22/2024 |
Title | |
Related Topics | Artificial neural networks, Artificial intelligence, Rotating disc computer storage media, Classification algorithms, Computational neuroscience |
Status | Grant |
Latest DeepMind News
Oct 25, 2024
An unprecedented 80 percent of Americans, according to a recent Gallup poll, think the country is deeply divided over its most important values ahead of the November elections. The general public’s polarization now encompasses issues like immigration, health care, identity politics, transgender rights, or whether we should support Ukraine. Fly across the Atlantic and you’ll see the same thing happening in the European Union and the UK. To try to reverse this trend, Google’s DeepMind built an AI system designed to aid people in resolving conflicts. It’s called the Habermas Machine after Jürgen Habermas, a German philosopher who argued that an agreement in a public sphere can always be reached when rational people engage in discussions as equals, with mutual respect and perfect communication. But is DeepMind’s Nobel Prize-winning ingenuity really enough to solve our political conflicts the same way they solved chess or StarCraft or predicting protein structures ? Is it even the right tool? Philosopher in the machine One of the cornerstone ideas in Habermas’ philosophy is that the reason why people can’t agree with each other is fundamentally procedural and does not lie in the problem under discussion itself. There are no irreconcilable issues—it’s just the mechanisms we use for discussion are flawed. If we could create an ideal communication system, Habermas argued, we could work every problem out. “Now, of course, Habermas has been dramatically criticized for this being a very exotic view of the world. But our Habermas Machine is an attempt to do exactly that. We tried to rethink how people might deliberate and use modern technology to facilitate it,” says Christopher Summerfield, a professor of cognitive science at Oxford University and DeepMind’s staff scientist who worked on the Habermas Machine. The Habermas Machine relies on what’s called the caucus mediation principle. This is where a mediator, in this case the AI, sits through private meetings with all the discussion participants individually, takes their statements on the issue at hand, and then gets back to them with a group statement, trying to get everyone to agree with it. DeepMind’s mediating AI plays into one of the strengths of LLMs, which is the ability to briefly summarize a long body of text in a very short time. The difference here is that instead of summarizing one piece of text provided by one user, the Habermas Machine summarizes multiple texts provided by multiple users, trying to extract the shared ideas and find common ground in all of them. But it has more tricks up its sleeve than simply processing text. At a technical level, the Habermas Machine is a system of two large language models. The first is the generative model based on the slightly fine-tuned Chinchilla, a somewhat dated LLM introduced by DeepMind back in 2022. Its job is to generate multiple candidates for a group statement based on statements submitted by the discussion participants. The second component in the Habermas Machine is a reward model that analyzes individual participants’ statements and uses them to predict how likely each individual is to agree with the candidate group statements proposed by the generative model. Once that’s done, the candidate group statement with the highest predicted acceptance score is presented to the participants. Then, the participants write their critiques of this group statement, feed those critiques back into the system which generates updated group's statements and repeats the process. The cycle goes on till the group statement is acceptable to everyone. Once the AI was ready, DeepMind’s team started a fairly large testing campaign that involved over five thousand people discussing issues such as “should the voting age be lowered to 16?” or “should the British National Health Service be privatized?” Here, the Habermas Machine outperformed human mediators. Scientific diligence Most of the first batch of participants were sourced through a crowdsourcing research platform. They were divided into groups of five, and each team was assigned a topic to discuss, chosen from a list of over 5,000 statements about important issues in British politics. There were also control groups working with human mediators. In the caucus mediation process, those human mediators achieved a 44 percent acceptance rate for their handcrafted group statements. The AI scored 56 percent. Participants usually found the AI group statements to be better written as well. But the testing didn’t end there. Because people you can find on crowdsourcing research platforms are unlikely to be representative of the British population, DeepMind also used a more carefully selected group of participants. They partnered with the Sortition Foundation, which specializes in organizing citizen assemblies in the UK, and assembled a group of 200 people representative of British society when it comes to age, ethnicity, socioeconomic status etc. The assembly was divided into groups of three that deliberated over the same nine questions. And the Habermas Machine worked just as well. The agreement rate for the statement “we should be trying to reduce the number of people in prison” rose from a pre-discussion 60 percent agreement to 75 percent. The support for the more divisive idea of making it easier for asylum seekers to enter the country went from 39 percent at the start to 51 percent at the end of discussion, which allowed it to achieve majority support. The same thing happened with the problem of encouraging national pride, which started with 42 percent support and ended at 57 percent. The views held by the people in the assembly converged on five out of nine questions. Agreement was not reached on issues like Brexit, where participants were particularly entrenched in their starting positions. Still, in most cases, they left the experiment less divided than they were coming in. But there were some question marks. The questions were not selected entirely at random. They were vetted, as the team wrote in their paper, to “minimize the risk of provoking offensive commentary.” But isn’t that just an elegant way of saying, 'We carefully chose issues unlikely to make people dig in and throw insults at each other so our results could look better?' Conflicting values “One example of the things we excluded is the issue of transgender rights,” Summerfield told Ars. “This, for a lot of people, has become a matter of cultural identity. Now clearly that’s a topic which we can all have different views on, but we wanted to err on the side of caution and make sure we didn’t make our participants feel unsafe. We didn’t want anyone to come out of the experiment feeling that their basic fundamental view of the world had been dramatically challenged.” The problem is that when your aim is to make people less divided, you need to know where the division lines are drawn. And those lines, if Gallup polls are to be trusted, are not only drawn between issues like whether the voting age should be 16 or 18 or 21. They are drawn between conflicting values. The Daily Show’s Jon Stewart argued that, for the right side of the US’s political spectrum, the only division line that matters today is “woke” versus “not woke.” Summerfield and the rest of the Habermas Machine team excluded the question about transgender rights because they believed participants’ well-being should take precedence over the benefit of testing their AI’s performance on more divisive issues. They excluded other questions as well like the problem of climate change. Here, the reason Summerfield gave was that climate change is a part of an objective reality—it either exists or it doesn’t, and we know it does. It’s not a matter of opinion you can discuss. That’s scientifically accurate. But when the goal is fixing politics, scientific accuracy isn’t necessarily the end state. If major political parties are to accept the Habermas Machine as the mediator, it has to be universally perceived as impartial. But at least some of the people behind AIs are arguing that an AI can’t be impartial. After OpenAI released the ChatGPT in 2022, Elon Musk posted a tweet, the first of many, where he argued against what he called the “woke” AI. “The danger of training AI to be woke—in other words, lie—is deadly,” Musk wrote . Eleven months later, he announced Grok, his own AI system marketed as “anti-woke.” Over 200 million of his followers were introduced to the idea that there were “woke AIs” that had to be countered by building “anti-woke AIs”—a world where the AI was no longer an agnostic machine but a tool pushing the political agendas of its creators. Playing pigeons’ games “I personally think Musk is right that there have been some tests which have shown that the responses of language models tend to favor more progressive and more libertarian views,” Summerfield says. “But it’s interesting to note that those experiments have been usually run by forcing the language model to respond to multiple-choice questions. You ask ‘is there too much immigration’ for example, and the answers are either yes or no. This way the model is kind of forced to take an opinion.” He said that if you use the same queries as open-ended questions, the responses you get are, for the large part, neutral and balanced. “So, although there have been papers that express the same view as Musk, in practice, I think it’s absolutely untrue,” Summerfield claims. Does it even matter? Summerfield did what you would expect a scientist to do: He dismissed Musk’s claims as based on a selective reading of the evidence. That’s usually checkmate in the world of science. But in the world politics, being correct is not what matters the most. Musk was short, catchy, and easy to share and remember. Trying to counter that by discussing methodology in some papers nobody read was a bit like playing chess with a pigeon. At the same time, Summerfield had his own ideas about AI that others might consider dystopian. “If politicians want to know what the general public thinks today, they might run a poll. But people’s opinions are nuanced, and our tool allows for aggregation of opinions, potentially many opinions, in the highly dimensional space of language itself,” he says. While his idea is that the Habermas Machine can potentially find useful points of political consensus, nothing is stopping it from also being used to craft speeches optimized to win over as many people as possible. That may be in keeping with Habermas’ philosophy, though. If you look past the myriads of abstract concepts ever-present in German idealism, it offers a pretty bleak view of the world. “The system,” driven by power and money of corporations and corrupt politicians, is out to colonize “the lifeworld,” roughly equivalent to the private sphere we share with our families, friends, and communities. The way you get things done in “the lifeworld” is through seeking consensus, and the Habermas Machine, according to DeepMind, is meant to help with that. The way you get things done in “the system,” on the other hand, is through succeeding—playing it like a game and doing whatever it takes to win with no holds barred, and Habermas Machine apparently can help with that, too. The DeepMind team reached out to Habermas to get him involved in the project. They wanted to know what he’d have to say about the AI system bearing his name. But Habermas has never got back to them. “Apparently, he doesn’t use emails,” Summerfield says. Science, 2024. DOI: 10.1126/science.adq2852
DeepMind Frequently Asked Questions (FAQ)
When was DeepMind founded?
DeepMind was founded in 2010.
Where is DeepMind's headquarters?
DeepMind's headquarters is located at 5 New Street Square, London.
What is DeepMind's latest funding round?
DeepMind's latest funding round is Acquired.
Who are the investors of DeepMind?
Investors of DeepMind include Google, Founders Fund, Horizons Ventures, Elon Musk and Jaan Tallinn.
Who are DeepMind's competitors?
Competitors of DeepMind include Rhino.ai, Huma, xAI, BioMap, RealAI and 7 more.
Loading...
Compare DeepMind to Competitors
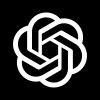
OpenAI is an artificial intelligence research and deployment company focused on ensuring that AI benefits all of humanity. The company's main offerings include developing AI technologies with a commitment to safety, alignment with human values, and broad societal benefits. OpenAI's products and services are designed to address global challenges and promote the equitable distribution of AI advantages. It was founded in 2015 and is based in San Francisco, California.
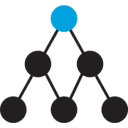
Numenta is a company that focuses on artificial intelligence technology within the tech industry. The company offers a platform for intelligent computing, providing neuroscience-based AI solutions that enable efficient, scalable, and secure deployment of Large Language Models (LLMs) within a user's infrastructure. Numenta primarily serves sectors that require advanced AI capabilities, such as the gaming industry, AI innovation sectors, and companies requiring large document processing. It was founded in 2005 and is based in Redwood City, California.
Google AI focuses on the development and application of artificial intelligence across various sectors. The company offers a range of AI-driven products and services, including large language models, text-to-image technology, and quantum computing research, aimed at enhancing scientific discovery and societal benefits. Google AI primarily sells to sectors that require advanced AI solutions, such as the technology and research industries. It was founded in 2017 and is based in Mountain View, California. Google AI operates as a subsidiary of Google.
IBM Watson Group focuses on artificial intelligence and hybrid cloud solutions within the technology sector. The company offers generative AI experiences, AI-powered cybersecurity, and post-quantum cryptography standards, as well as services in AI and machine learning, analytics, compute and servers, databases, DevOps, IT automation, quantum computing, and security and identity. IBM Watson Group's products and services are primarily utilized by various sectors including the sports industry, cybersecurity, and enterprise IT infrastructure. It is based in New York, New York. IBM Watson Group operates as a subsidiary of IBM.

Verily operates as a precision health company in the healthcare and technology sectors. The company offers solutions that accelerate clinical research and enable more personalized care, leveraging data science, artificial intelligence, and cloud-based analytics. Its services are primarily utilized in the healthcare industry. It was formerly known as Google Life Sciences. It was founded in 2015 and is based in South San Francisco, California.

Anthropic is an AI safety and research company that specializes in developing advanced AI systems. The company's main offerings include AI research and products that prioritize safety, with a focus on creating conversational AI assistants for enterprise use. Anthropic primarily serves sectors that require reliable and interpretable AI technology. It was founded in 2021 and is based in San Francisco, California.
Loading...