We examine how big tech and startups are developing new computing methods that could advance AI.
What you need to know:
- Increasing computational demands and energy consumption of LLMs are driving the exploration of novel computing approaches like biological, neuromorphic, photonic, and quantum computing.
- Corporate VCs and big tech are getting involved, signaling the AI arms race is heating up as companies looking for next-generation computing solutions for AI workloads.
- While still nascent, new computing approaches offer unique advantages in processing speed, energy efficiency, and potential AI capabilities.
Specialized chips called graphical processing units (GPUs) are essential for AI computing today because they can handle many small sub-tasks simultaneously. ChatGPT reportedly relies on nearly 300,000 GPUs.
But GPUs are also power-hungry, and it will eventually become difficult to squeeze more performance out of them as we begin to run into the physical limits of how small and efficient traditional transistors can become.
The desire to run increasingly complex AI workloads has led investors and startups to explore new energy-efficient processors and computing approaches that could be more performant for AI tasks — and make it more feasible to run intensive AI systems that can push past the reasoning limitations LLMs currently face.
In this brief, we break down 4 novel computing approaches that show promise for AI workloads, as highlighted in our recent market map: photonic, neuromorphic, biological, and quantum computing.
Source: CB Insights — AI Computing Hardware Market Map
As AI applications proliferate, enterprises with data center operations and corporate investors more broadly should pay particular attention to developments here.
Want to see more research? Join a demo of the CB Insights platform.
If you’re already a customer, log in here.
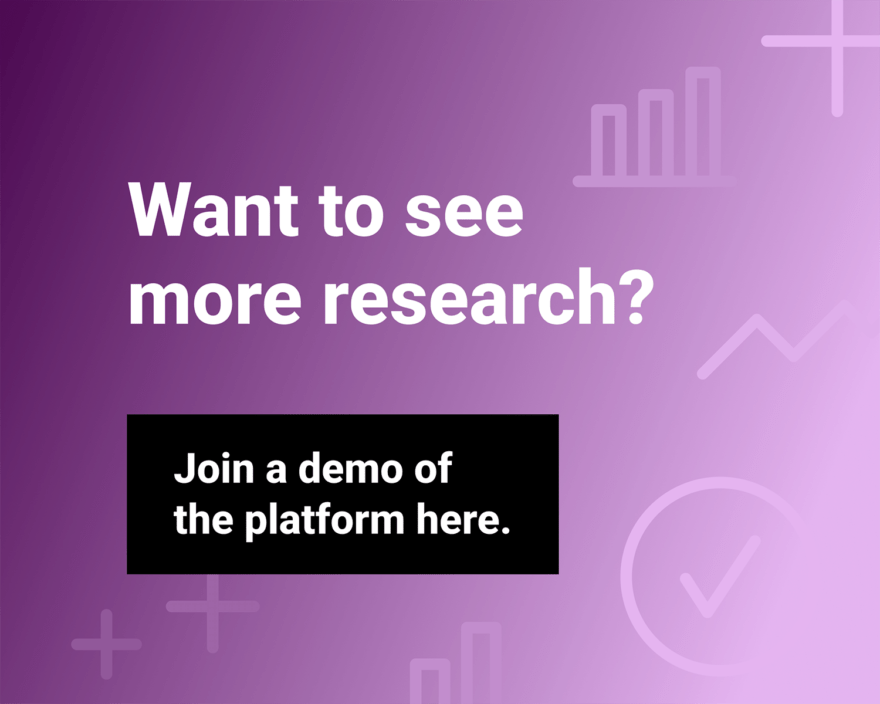