
Kriya
Founded Year
2011Stage
Line of Credit - III | AliveTotal Raised
$799.45MLast Raised
$63.38M | 9 mos agoMosaic Score The Mosaic Score is an algorithm that measures the overall financial health and market potential of private companies.
+8 points in the past 30 days
About Kriya
Kriya specializes in business-to-business payment solutions within the financial services sector. The company offers trade credit options for eCommerce checkouts, multichannel payment systems, and flexible invoice finance services to bridge cash flow gaps. Kriya primarily serves businesses looking to modernize their payment processes and their working capital management. Kriya was formerly known as MarketFinance. It was founded in 2011 and is based in London, United Kingdom.
Loading...
ESPs containing Kriya
The ESP matrix leverages data and analyst insight to identify and rank leading companies in a given technology landscape.
The invoice finance market allows businesses to access funds associated with unpaid invoices. Through APIs and user-friendly software, these platforms connect companies needing immediate cash flow with investors or financial institutions willing to advance funds against outstanding invoices. This technology-driven approach simplifies invoice processing, enhances transparency, and speeds up funding…
Kriya named as Outperformer among 15 other companies, including Ramp, C2FO, and Funding Xchange.
Loading...
Research containing Kriya
Get data-driven expert analysis from the CB Insights Intelligence Unit.
CB Insights Intelligence Analysts have mentioned Kriya in 2 CB Insights research briefs, most recently on May 20, 2022.
Expert Collections containing Kriya
Expert Collections are analyst-curated lists that highlight the companies you need to know in the most important technology spaces.
Kriya is included in 5 Expert Collections, including Fintech 100.
Fintech 100
498 items
250 of the most promising private companies applying a mix of software and technology to transform the financial services industry.
Digital Lending
2,271 items
This collection contains companies that provide alternative means for obtaining a loan for personal or business use and companies that provide software to lenders for the application, underwriting, funding or loan collection process.
SMB Fintech
1,231 items
Payments
3,034 items
Companies in this collection provide technology that enables consumers and businesses to pay, collect, automate, and settle transfers of currency, both online and at the physical point-of-sale.
Fintech
13,413 items
Excludes US-based companies
Latest Kriya News
Oct 18, 2024
npj climate and atmospheric science Abstract Hydrological connectivity is crucial for understanding water-ecosystem dynamics, as it serves as a key link between different landscape units. However, the variability of hydrological connectivity in Central Asia remains unexplored, which poses challenges to a comprehensive understanding of ecohydrological processes. This study investigates the spatiotemporal patterns and driving mechanisms of hydrological connectivity in the Tarim River Basin (TRB), Central Asia, from 1990 to 2020, employing a novel approach that integrates remote sensing and reanalysis data. The results indicate an increasing trend in the hydrological connectivity index (HCI), with approximately 60% of the TRB exhibiting significant increases. Climate change exerts the greatest direct (0.59) and total (0.64) effects on HCI, with potential evapotranspiration (19.2%) and temperature (12.6%) being the dominant factors. In mountainous regions, climate change (0.65) is the primary driver, while human activities have a greater impact in the plains (−0.27). These findings offer a new framework for studying ecohydrological processes in arid regions. Introduction Hydrological connectivity plays a distinctive and crucial role in biodiversity conservation, geochemical cycling, and human social development 1 . It facilitates the movement and exchange of water, as well as water-mediated organisms, sediments, organic matter, nutrients, and energy in water patches 2 , thereby regulating the processes from water source to sink. This regulatory effect is particularly important in arid regions, where hydrological connectivity establishes and sustains dryland ecosystems by controlling the distribution of water resources between bare land and vegetation patches 3 . Consequently, hydrological connectivity is widely recognized as a key driver of both structural and functional variations in freshwater ecosystems 4 . The study of hydrological connectivity has emerged as a prominent research focus in hydrology, geomorphology, and geography 2 , 5 , 6 . Much of the research has concentrated on how to conceptually and empirically utilize hydrological connectivity to understand complex environmental systems 5 , 7 , 8 , significantly advancing the hydrological connectivity-related knowledge base. Studies have demonstrated that hydrological connectivity is an effective framework for interpreting water redistribution processes, which dominate the exchange of material energy in the water medium, which controls hydrological processes between sparsely vegetated landscapes and dryland 9 , and which affects the growth of vegetation 10 , 11 . Thus, hydrological connectivity can serve as an important indicator for detecting transitions and degradation thresholds in dryland systems 12 . In addition, the effects of hydrologic connectivity on river water quality 4 , 13 , runoff 7 , 8 , and biodiversity 14 , 15 have been widely scrutinized by researchers. It has been found that hydrological connectivity can drive extremes and high variability in vegetation productivity and water quality in arid and semi-arid ecosystems 10 , 13 , 16 , and its dynamics may challenge the persistence of a globally endemic fish fauna 14 , 15 . Moreover, the effects of human activities (i.e., dam construction, water flow regulation and diversion, and land management) 15 , 17 , 18 and natural factors (i.e., climate, vegetation, and topography) 2 , 14 , 19 on hydrological connectivity should also be emphasized. These anthropogenic and natural factors alter the underlying surface topography, which in turn significantly affects regional hydrological connectivity 20 . This process can induce substantial changes in landscape structure and function, triggering regional ecosystem responses 21 . Such changes are particularly concerning in arid regions, due to the fragile ecology and vulnerability of these locations to disturbances from external factors 12 . Even the minor climate change, such as gradual warming or reduced precipitation, will affect the frequency and severity of hydrological drought 14 . Yet it is worth noting that the response of hydrological connectivity to climate change and human activities is still unclear. Therefore, to enhance our understanding of the mediating role of hydrological connectivity in dryland ecohydrological processes, further research on hydrological connectivity in dyland rivers and its driving factors in essential. Such research is crucial for predicting how these processes will evolve under fluctuating conditions and increasing anthropogenic activities 22 . In recent years, the loss of hydrological connectivity has emerged as a global threat to rivers 23 . Only 37% of the world’s rivers longer than 1000 kilometers remain free-flowing along their entire length, and only 23% maintain uninterrupted flow into the ocean 24 . In arid regions, the loss of river connectivity has been exacerbated by water resource scarcity and ecosystem fragility 25 . Despite these critical changes to vulnerable ecosystems, few studies utilized observational data to quantify hydrological connectivity at the network and watershed scales of inland rivers in arid regions 26 . Furthermore, most studies have assessed structural hydrological connectivity, which is determined by the spatial pattern of the landscape, referring to process-based connectivity 6 , and usually changes on long time scales 19 , 27 , 28 . It depends largely on the interactions among multiple components of flow state as well as watershed geomorphology 20 . Thus, hydrological processes need to be linked to internal geomorphological changes when targeting hydrological connectivity studies in large-scale watersheds 29 , yet this presents a significant challenge in the assessment of functional hydrologic connectivity. Traditional functional hydrological connectivity assessment methods mainly include the dendritic connectivity index (DCI) 30 , water level difference 31 , and the comprehensive evaluation index system 24 . These and earlier assessment methods have advanced our understanding of hydrological connectivity, though their research methods differ in focus. For instance, the DCI assessment method, which is widely used to evaluate barrier-dominated river functional connectivity, characterizes functional hydrological connectivity by the length of a river segment separated by barriers 30 . However, because water forms the foundation of hydrological connectivity, dynamic changes in surface water bodies maintain connectivity by creating temporary habitats and providing opportunities for ephemeral dispersion 32 . Therefore, there is an urgent need to find a way to integrate these changes into the assessment of hydrologic connectivity. This study proposes an improved method for hydrological connectivity assessment. The novel approach quantifies hydrological connectivity and its change characteristics at both river network and basin scales, using observational data from 1990 to 2020. This establishes a framework for investigating hydrological connectivity in arid regions. The Tarim River Basin (TRB) in Central Asia was selected as the study area. The TRB is a typical inland river basin characterized by significant ecological vulnerability and severe water resource shortage, making it a priority area for soil erosion control in China. While the TRB is often used as a model basin in arid Central Asia for eco-hydrology studies 33 , 34 , 35 . However, few studies have yet been conducted to quantify the hydrological connectivity in this region. The three main areas of research focus in this study are: 1) proposing an improved hydrological connectivity index (HCI) and identifying spatial and temporal changes in the hydrological connectivity of the TRB from 1990 to 2020; 2) quantifying the contributions of natural and anthropogenic factors to hydrological connectivity variability at the regional scale; and 3) assessing the direct and indirect impact pathways of dominant factors on HCI variability. The study results are expected to be used as a research paradigm for assessing the hydrological connectivity of inland river basins in arid regions and as scientific support for developing soil and water management strategies. Results Spatio-temporal dynamic analysis of hydrological connectivity in the TRB The hydrological connectivity of the TRB exhibited a clear increasing trend (Fig. 1a ), with the HCI rising at a rate of 0.08/a during the study period and a multiannual average of −5.77. Fluctuations in the HCI were more pronounced in the 1990s (SD = 1.05) but showed steady growth after 2000 (Fig. 1a ). The proportion of streams with high HCI also gradually increased over time (Supplementary Fig. 1 ), and the HCI spatially was lower in the mountain of TRB (TRB-M) than in the plain (TRB-P) (Fig. 1b ). This is primarily due to the process of river formation (rivers are formed in the TRB-M), where rivers are disconnected and water flux is lower. It is worth noting that hydrological connectivity increases as runoff pools into the plains 36 . On the other hand, the wide distribution of artificial drainage systems in the TRB-P may also contribute to the phenomenon. As confirmed by the hot spot analysis (Supplementary Fig. 2 ), the hot spots (high-value areas) of the HCI are concentrated in the hinterland (plain) of the basin, while the cold spots (low-value areas) are concentrated in the high-altitude mountain. Fig. 1: Distribution of HCI in the Tarim River Basin (TRB) from 1990 to 2020. a Annual distribution of the HCI and trend analysis of interannual average values in the TRB from 1990 to 2020 (statistics at 30 × 30 m grid scale), each boxplot represents the distribution of HCI at the TRB grid point scale for that year; the wavy line is fitted to the annual average HCI from 1990 to 2020, the dotted line represents the fitted line of annual average HCI for different time periods, where blue represents 1990 to 2000, orange 2000 to 2010 and green 2010 to 2020. b HCI in the TRB in ten sub-basins in the mountains and plains, where green represents the mountains and purple represents the plains. c Annual average HCI of sub-basins and trend changes, dots represent the annual average HCI value in each basin. The Kaikong River Basin exhibits higher HCI in the mountains compared to the plains (Fig. 1b ). This is largely due to the presence of the Bayinbuluk grassland, the largest grassland in southern Xinjiang, which spans the mountains region. The extensive vegetation cover in this area trap water and sediment, reducing hydrological connectivity. Meanwhile, the widespread croplands in the lower reaches of the basin consume substantial water resources, and annual ecological water transfer projects are implemented from the lower reaches of the Kongque River to the lower Tarim River 33 . These anthropogenic activities significantly reduce hydrological connectivity in the plains compared to the mountain regions. Changes in hydrological connectivity at the basin scale reveal significant spatial heterogeneity. During the study period, HCI showed an increasing trend in all the basins, with the Dinar River Basin, the Weigan River Basin, and the Tarim River mainstem showing the most significant increases, at rates of 0.14/a, 0.12/a, and 0.12/a, respectively (Fig. 1c ). These increases in connectivity are also related to a series of ecological water transfer projects in the TRB. In addition, the HCI of the Kashgar, Kriya, and Qarqan river basins is low and rising slowly at the rate of 0.05/a, 0.03, and 0.04, respectively. This is due to the loss of surface hydraulic relationships between the Kashgar, Kriya, and Qarqan rivers and the mainstem of the Tarim River 36 , which has disconnected the rivers in the region. The result is the destruction of the integrity of the regional ecosystem and a slowing of the hydrological connectivity restoration. Meanwhile, HCI of watersheds increases spatially with latitude and longitude. The connectivity is lower in the southwest (Kashgar, Yarkand and Kriya river basins) than in the northeast (Aksu, Weigan, Dinar, and Qarqan river basins) of the TRB (Fig. 1c ). This is related to the topographic setting of the region. The entire basin exhibits a geomorphic pattern that converges to the northeast; water resources flow to the northeast in accordance with the topographic pattern, which results in higher HCI in watersheds located in northeast basin. The results of our statistical analysis (Hydrobasin level 8) show that about 60% of the basin exhibited a significant increasing trend in the HCI ( | Z | ≥ 1.96 & SHCI > 0) (Fig. 2 a, b ) at a rate of increase ranging from 0 to 0.2. The areas of increased HCI are mainly distributed in the Dinar River Basin as well as in the mainstem of the Tarim River. Meanwhile, variations in the HCI (∆HCI) are more prominent in the plains, with the highest ∆HCI reaching 7.6 during the study period (Fig. 2c ). The main reasons for the reduction in hydrological connectivity in these locations are the significant decrease in the HCI in the oasis area near the Kongque River and the lower reaches of the mainstem, the relatively extensive human activities in the oasis area, and the widespread distribution of desert land in the lower reaches of the mainstem. Fig. 2: Variation of HCI in the TRB from 1990 to 2020. a Results of interannual variation trend and significance analysis of the HCI in the TRB from 1990 to 2020 (Hydrobasin level 8). b Statistics of HCI variation trend, the circles show the percentage of area in different HCI trends. The inner-ring color corresponds to the left-side color and statistics, and the outer-ring color corresponds to the right-side color and statistics. c Spatial distribution of HCI variation (△HCI) from 1990 to 2020. Dominant factors of the HCI Using the random forest model (RFM), we calculated the normalized percent increase in mean squared error (%InMSE) of the potential drivers to estimate their contribution to the HCI, as shown in Fig. 3 . We found that the biggest contributors in the TRB are potential evapotranspiration (PET), WA, temperature (TEM), Normalized Difference Vegetation Index (NDVI), elevation (ELE), topographic wetness index (TWI), human foot print (HFP), slope, and precipitation (PRE) (Fig. 3a ). Specifically, PET (19.2%), WA (12.9%), and TEM (12.6%) each contributed over 10% to HCI variations, while snow water equivalent (SWE) (end of month) and land use and land cover change (LUCC) contributed less than 5%. In the TRB-M, we found that TEM contributed the most to the HCI, with contributions up to 23% (Fig. 3a ). However, in the TRB-P, we found that the dominant factor was WA, and the contributions of climate factors were much less than in the TRB-M. This is because in the TRB-M, climate warming significantly increases glacial snowmelt, which increases regional water availability and promotes hydrological connectivity 34 . Fig. 3: Contribution of potential drivers to HCI. a Relative importance of potential drivers of the TRB, TRB-M, and TRB-P output from RFM on the HCI, with the relative contribution expressed as the normalized percent increase in mean squared error (%InMSE). b Importance of potential driver output from RFM on the watershed, with normalized %InMSE exceeding 10%. c Boxplot showing the importance of potential factors on the basin. Regionally, WA made a larger contribution to the HCI across all watersheds (Fig. 3 b, c ). At high latitudes, the dominant factors impacting the HCI were TEM, NDVI, HFP, and TWI, whereas at low latitudes, topographical factors (ELE and slope) dominated (Fig. 3b ). This is because the TRB originates in the Pamir Plateau and the Kunlun Mountains at low latitudes (Fig. 6b ), where significant topographical variations make topographic factors the primary influence on HCI variations. Influencing pathways of dominant factors on HCI variations Based on the results of RFM, the final Partial Least Squares Structural Equation Modeling (PLS-SEM) fitting model of the TRB is shown in Fig. 4 . As can be seen, the goodness of fit (GOF) of the PLS-SEM model is 0.57, 0.52, and 0.4 for the TRB, TRB-M, and TRB-P, respectively. These natural and anthropogenic drivers explained 31% of the HCI in the basin (Fig. 4a ) and all of the direct influence paths on the HCI passed the significance test (P < 0.05). Among them, climate factors demonstrated the greatest direct influence, with a path coefficient of 0.59. Additionally, climate can indirectly influence the HCI through hydrology (TEM/PET → WA → HCI) and vegetation (TEM/PET → NDVI → HCI) (Supplementary Table 1 ). The largest total influence is thus from climatic features, with an influence coefficient as high as 0.64 (Fig. 4d ). This is because climate change can substantially impact regional eco-hydrological processes, which in turn leads to changes in hydrological connectivity. In particular, increased temperatures cause accelerated melting of glacial snow and increased regional water availability 34 , while increased evapotranspiration results in decreased hydrological connectivity due to increased water depletion. Therefore, we conclude that the increase in hydrological connectivity of the TRB is mainly caused by the increased temperature. Fig. 4: PLS-SEM is used to analyze path relationships of indicators and the HCI during 2000-2020. a The result of PLS-SEM in TRB. b The result of PLS-SEM in mountains of TRB (TRB-M). c The result of PLS-SEM in plains of TRB (TRB-P). Where blue represents hydrology (HY), green represents climate (CT), orange represents human activities (HA), yellow represents topography (TP), and purple represents vegetation (VG), and line color corresponds to indicator color and line width is proportional to the influence coefficient. d Direct, indirect, and total influences of different factors on the HCI in the three areas. Note that * indicates significance at p < 0.05 levels, respectively, while “N.A.” means “Not Applicable”. A significant positive correlation was found between hydrological characteristics (path coefficient: 0.15, p < 0.05) and the HCI, whereas human activities and vegetation characteristics had a negative influence, with path coefficients of −0.12 and −0.1, respectively. The modification of the natural environment by human activities is more complex and can influence the HCI through multiple pathways. For example, anthropogenic activities affect the HCI by modifying topographic features (HFP → DEM/slope→HCI) or by consuming water resources (HPF → WA → HCI). Human activities can also increase or weaken the HCI by influencing climate change (HFP → TEM → HCI). Vegetation growth enhances surface roughness, improves sediment and water retention, and reduces hydrological connectivity 7 . It is worth noting that topographical factors in the TRB have the greatest indirect influence on the HCI, with a path coefficient of −0.54. Both natural and anthropogenic factors can affect water and sediment flow by altering topographic features 37 , while heterogeneity of climate (DEM → TEM → HCI) and vegetation characteristics (DEM → NDVI → HCI) under different topographic factors can indirectly affect the HCI. Across the TRB, potential drivers varied notably between mountainous and plains regions. This was especially true of the climate factor, which showed a path coefficient of 0.58 for the HCI in the mountains and 0.14 in the plains (Fig. 4 b, c ). The response of mountain ecosystems to climate change is more sensitive, with warming intensifying glacial meltwater and increasing hydrological connectivity 14 . Moreover, there is a major difference in the impact of human activities on the HCI, with path coefficients of −0.04 and −0.14 in the mountainous and plains regions, respectively. This situation is also attributed to the decline in hydrological connectivity due to the depletion of water resources by human activities and the concentration of anthropogenic areas mainly in the plains. The outcome is a greater impact of human activities on the plains than on the mountainous regions. Moreover, the path coefficients of topographic features on the HCI are different in the TRB-M (0.12) and TRB-P (−0.12). Possible reasons for this phenomenon are topographic features that lead to an increase in water rates and sediment exchange in mountains with steeper slopes, which can promote hydrologic connectivity. In the plains, on the other hand, as the change in elevation and slope slows down, water flow stops at zero slope and hydrological connectivity gradually decreases in the absence of external forces 7 . Discussion Hydrological connectivity is significantly influenced by various status components (e.g., frequency, duration, timing, rate of change, etc.) and fluvial geomorphology (e.g., channel and floodplain shape, size, gradient, sediment composition, location, etc.) 2 , 38 . Surface water sustains connectivity by providing temporary dispersal opportunities 21 , 32 . Remote sensing not only serves as the primary method for identifying surface water areas, but also provides a crucial basis for assessing hydrological connectivity 20 . Functional hydrological connectivity is positively correlated with both the frequency of surface water and its ability to traverse regional topography 29 . Based on the DCI 30 , functional hydrological connectivity can be conceptualized as the product of surface water frequency and topographic passage. Compared to previous methods 24 , 30 , 31 , the HCI relies on remote sensing observations, enabling the assessment of hydrological connectivity in data-deficient areas and increasing the diversity of spatial and temporal assessment scales. This is an important breakthrough for arid regions. This improved assessment methodology focuses on incorporating water body frequencies into the assessment process, which addresses the subjectivity of connectivity threshold values on the landscape 39 . Compared with DCI, HCI focuses on the integration of water frequency into the assessment of hydrological connectivity, rather than the proportion of river segments 6 , 30 . The water frequency provides a priori probability of the presence of water at each image element, which indicates the historical occurrence of water at that location 40 . The frequency of the water body can respond not only to the acquisition of water but also to the results of the exchange of the water body, which is the result of the influence of various environmental factors on hydrological processes 41 , 42 . So this makes it limited for HCI to reduce the impact of channel barriers on hydrological connectivity, even though the alteration of hydrological connectivity by these barriers is significant 17 , 43 . Nevertheless, the number of dams in arid regions is lower than in other regions. In the TRB, there are significantly fewer dams, and these are primarily located in the plains (Supplementary Fig. 3 ). Despite, there is still a need for further research on the significant impact of these barriers on hydrological connectivity in arid regions. A multi-scale assessment of hydrological connectivity in the TRB using the HCI reveals lower hydrological connectivity in the mountains compared to the plains (Fig. 1b ). This contrasts with the findings of Grill et al. 24 , who reported higher free-flowing capacity in mountain rivers in the TRB region. The discrepancy between results arises because Grill’s assessment primarily considered the impact of anthropogenic activities, such as barriers (dams and reservoirs), consumptive water use, road density, and urban areas, which led to a finding of lower hydrological connectivity in the plains where human activities are more frequent. River connectivity is also influenced by natural environmental factors 2 . In mountainous regions where rivers originate, channels are narrower and have lower water volume. As these rivers converge and flow into the plains, runoff increases, the channels widen, and hydrological connectivity is gradually enhanced 20 . Additionally, the construction of artificial canals in the plains will increase hydrological connectivity paths 27 . Our study incorporates indicators related to topography and hydrology, considering both anthropogenic and natural factors, including dynamic subsurface changes. Our findings indicate that climate change significantly influences regional hydrological connectivity (Fig. 3 ), which may be the primary reason for the observed differences. Hydrological connectivity can provide a new perspective for ecohydrological feedback studies and become a research hot spot in ecohydrology 44 . The connectivity of runoff formation areas is recognized as one of the main factors controlling sparsely vegetated landscapes 9 . Especially in arid regions, hydrological connectivity determines the process of water redistribution between bare land and vegetation patches 28 . Research by Norton et al. 10 demonstrated that hydrological connectivity drives extremes and high variability in vegetation productivity in arid and semi-arid ecosystems. The ability to transfer and exchange the water increases with higher hydrological connectivity, which in turn promotes vegetation growth. However, our study shows that the path coefficients of vegetation on the HCI are negative (Fig. 4 ). This is because vegetation patches, along with related woody debris and litter, increase surface roughness. Consequently, these areas exhibit greater resistance to flow compared to bare land 7 , 11 . The increased resistance enhances water and sediment retention capacity, while also affecting the transfer capacity of water and sediment and altering their transfer and transportation pathways, ultimately reducing hydrological connectivity 45 , 46 . An inverted U-shaped relationship between vegetation and hydrological connectivity was demonstrated 44 , i.e., increased hydrological connectivity promotes material-energy exchanges between water patches, which facilitates vegetation growth and then gradually reaches a balance. While the proportion of water surface increases with further increases in hydrological connectivity 13 , the expanded water area occupies space for vegetation growth, potentially destroying vegetation habitat and causing vegetation cover to decrease as connectivity grows 44 . In arid regions, the redistribution of water between bare land and vegetated areas enables the establishment and persistence of dryland ecosystems 12 . With precipitation too low to support the natural growth of vegetation, runoff is largely dependent on glacial snowmelt recharge 1 , 34 . As the climate warms, increased glacial snowmelt increases the runoff, contributing to enhanced hydrological connectivity (Fig. 4a & Fig. 5 ). To further examine the relationship between hydrological connectivity and surface runoff, we analyzed the relationship between the HCI and runoff by selecting three major rivers (Kaikong, Yarkand, and Hotan) in the TRB (Fig. 5 ). Our results showed a significant positive correlation, which means that as the runoff increased, the HCI also increased. These findings align with previous studies 8 , 26 , which found that when hydrological connectivity was low, runoff generation efficiency increased with rising connectivity. However, at higher levels of connectivity, it began to inhibit runoff and precipitation production efficiency. Fig. 5: Correlation between runoff and HCI. a Correlations between runoff and the HCI in the Kaikong b Yarkand, and c Hotan river basins; Shaded areas are 95% confidence intervals. d Boxplots of the IC for different runoff ranges in the Kaikong e Yarkand, and f Hotan river basins. In arid regions, minor changes in vegetation patterns can significantly alter surface hydrological connectivity and affect ecosystem functioning 3 , 14 , 28 . Irrigation in arid areas is mainly dependent on groundwater and a significant increase in the irrigated area in the study area 35 , 47 , which will increase the exploitation of groundwater resources and raise the contradiction between ecological water usage and agricultural water usage 25 , 35 . This will result in reduced natural groundwater recharge and altered surface runoff patterns 36 , and will affect the connectivity between surface water and groundwater, as well as alter the landscape pattern of surface vegetation. In this scenario, the redistribution of water between bare land and vegetation patches is disrupted, resulting in a further reduction in the water available to sustain ecosystem functionality 12 and posing a threat to the stability of arid land ecosystems. On the other hand, however, irrigation will to some extent increase soil moisture, increase surface vegetation cover, and alter surface hydrological connectivity 12 , 46 . Meanwhile, improvements in irrigation technology and the implementation of ecological water conveyance projects could contribute to maintaining or improving hydrological connectivity to a certain extent 27 . As the hydrological connectivity of the TRB increasing (Fig. 1a ), we should further enhance the ecohydrological response to this phenomenon. This is important for understanding the soil-water cycle and ecohydrological processes in arid regions. In conclusion, the ecohydrological effects of hydrological connectivity also need to be further explored to provide scientific support for the sustainable development of arid regions. Methods Selection of typical inland river basin in arid Central Asia The Tarim River Basin is located in the hinterland of Central Asia (Fig. 6a ). The annual average precipitation from 1989 to 2015 was 17.4–42.0 mm, while the annual evaporation was 2500–3000 mm. The basin consists of nine major headwaters (Kaikong, Dina, Qarqan, Weigan, Aksu, Kashgar, Yarkant, Hotan, and Keriya River) with 144 tributaries (Fig. 6a ) 35 , giving it a watershed area of ~1.02 × 106 km2 and a total surface runoff of ~3.98 × 1010 m3. The runoff mainly consists of snow and ice meltwater in the mountains, forest precipitation in the mid-mountain belt, and bedrock fissure water in the low-mountain belt. Fig. 6: Overview of the Tarim River Basin (TRB). a Location of the TRB and sub-basin delineation. b Distribution of elevation, major rivers, and hydrological stations in the TRB. c Distribution of land use in the TRB in 2020. d Distribution of mountains (TRB-M) (>1500 m) and plains (TRB-P) (≤1500 m), with mountain shading superimposed on the background. The rivers in the map are classified according to the water system in HydroSHEDS (Hydrobasin level 8). Of the nine headwater streams of the Tarim River, only the Aksu, the Hotan, and the Yarkand rivers remain in surface hydraulic connection with the mainstem (Fig. 6b ), and of these three, the Aksu alone maintains a perennial surface hydraulic connection. The Alsu originates in the Tianshan Mountains to the north and comprises the main source of recharge for the Tarim River. The Hotan and Yarkand rivers only briefly recharge the mainstem, and only during periods of abundant water; otherwise, they remain disconnected from it. In addition, the Kongque River is connected to the lower reaches of the Tarim River mainstem through the Kuta Main Canal at the lower reaches. The canal also provides one of the main routes for ecological water transfer in the lower reaches of the Tarim. At the center of the basin is the Taklamakan Desert (Fig. 6c ), the second-largest living sand sea in the world. To the northeast, the Tianshan Mountains are characterized by glaciers, grassland, and desert areas, while the plains are dominated by a mixture of farmland oases and arid desert. Surface water bodies account for less than 5%, which limits local ecological protection and agricultural development (Supplementary Fig. 4 ). Elevation higher than 1500 m is classified as a high mountain (TRB-M) and runoff-generating area, while elevation lower than 1500 m is designated as the plain (TRB-P) and runoff-consuming area (Fig. 6d ). Datasets The elevation (ELE) data for this study come from Version 3 (V003) of the Advanced Spaceborne Thermal Emission and Reflection Radiometer (ASTER) Global Digital Elevation Model (GDEM) dataset. The ASTER GDEM data product, which we based on ASTER data calculations covering the land surface between 83°N and 83°S, is the only global land surface high-resolution elevation imagery data source currently available. The latest version, V003, has a spatial resolution of 1 arc second (~30 meters) and the data are sliced into 1° × 1° chunks. The data is available at the Geospatial Data Cloud ( https://www.gscloud.cn , accessed on 1 September 2023). We also calculated the slope and topographic wetness index (TWI) based on this dataset for attribution analysis. Annual data for land use and land cover change (LUCC) from 1985 to 2020 was interpreted by Yang and Huang 48 using 335,709 Landsat images with a spatial resolution of 30 m ( https://zenodo.org/record/5210928 , accessed on 1 September 2023). The accuracy of the LUCC was stable and satisfactory, with an average overall accuracy of 79.30% ± 1.99%. The data classified the land/water area in the TRB into nine categories (cropland, forestland, shrubland, grassland, water, snow/ice, barren land, impervious and wetland), with the percentage of forest/shrub area being extremely low (about 0.2% of forest area and ≤ 0.001% of shrub area). Weighting factors were calculated according to the Manning coefficients corresponding to the land-use types for the calculation of sediment connectivity indices. Meteorological data come from the Terra Climate (TC) dataset ( http://www.climatologylab.org/ , accessed on 1 September 2022), from which we chose the following variables: precipitation (PRE), snow water equivalent (SWE) (end of month), temperature (TEM), and potential evapotranspiration (PET). The TC dataset, which compiles global land surface monthly-scale climate data covering 1958–2022, is a high-precision climate dataset with a spatial resolution of 4 km (1/24 degrees) 49 . It combines the high spatial resolution of the WorldClim dataset with the lower spatial resolution of the CRU Ts4.0 and the Japanese 55-year Reanalysis (JRA55) meteorological data. Normalized Difference Vegetation Index (NDVI) was obtained from the Terra Moderate Resolution Imaging Spectroradiometer (MODIS) Vegetation Indices (MOD13Q1), Version 6.1. The dataset is generated every 16 days at a 250 m spatial resolution as a Level 3 product. The MOD13Q1 product provides the NDVI layer, which is referred to as the continuity index to the existing National Oceanic and Atmospheric Administration-Advanced Very High-Resolution Radiometer (NOAA-AVHRR)-derived NDVI. The MODIS NDVI product is computed from atmospherically corrected bi-directional surface reflectance that has been masked for water, clouds, heavy aerosols, and cloud shadows. The algorithm chooses the best available pixel value based on all the acquisitions from a 16-day period. The criteria used are low clouds, low viewing angle, and highest NDVI value. We downloaded the NDVI dataset from Google Earth Engine ( https://developers.google.com/earth-engine/datasets/catalog/MODIS_061_MOD13Q1 , accessed on November 2023) and used these data for identifying the dominant factors of the HCI and for impact pathway analysis. Human Foot Print (HFP) is an indicator that characterizes the pressure on the ecosystem caused by human-induced changes in ecological processes and natural landscapes ( https://doi.org/10.6084/m9.figshare.16571064 , accessed on November 2023). The indicators are widely used to characterize human activities in eco-hydrology-related studies 42 , 50 . Developed by Mu et al. 51 , the HFP dataset includes global data from 2000-2020 at a resolution of 1000 m and follows the methodology adopted by Sanderson et al. 52 and Venter et al. 53 , with seven variables(population density, nighttime lighting, farmland, pastureland, roads, railroads, and navigable waterways) reflecting different aspects of human pressures on the built environment. Water body detection The NDVI, the modified NDWI (mNDWI), and the Enhanced Vegetation index (EVI) were used to map the surface water body in the TRB. These methods are widely employed in water body identification 41 , 54 , 55 . The indices were calculated using cloud-free and snow-free Landsat imagery based on the following spectral bands and equations: $${\rm{mNDWI}}=\frac{{\rho }_{{Green}}-{\rho }_{{SWIR}}}{{\rho }_{{Green}}+{\rho }_{{SWIR}}}$$ (1) (2) $${\rm{EVI}}=2.5\times \frac{{\rho }_{{NIR}}-{\rho }_{{Red}}}{1.0+{\rho }_{{NIR}}+6.0{\rho }_{{Red}}-7.5{\rho }_{{Blue}}}$$ (3) where ρ Blue, ρ Green, ρ Red, ρ NIR, and ρ SWIR are the surface reflectance values of blue (0.45–0.52 μm), green (0.52–0.60 μm), red (0.63–0.69 μm), near-infrared (NIR) (0.77–0.90 μm), and shortwave-infrared (SWIR) (1.55–1.75 μm) bands, respectively, in the Landsat TM, ETM+ and OLI. Furthermore, because EVI < 0.1 can eliminate vegetation pixels or mixed pixels of water and vegetation, either mNDWI > EVI or mNDWI > NDVI and EVI < 0.1 was utilized as the standard for identifying the pixels with a stronger water signal than vegetation signal. Only those pixels that meet the above standards are classified as water pixels, while the rest are classified as non-water pixels. For each landsat pixel, the annual water frequency (WF) was calculated using the following formula: $$f\left({\rm{y}}\right)=\frac{1}{{N}_{y}}\mathop{\sum}_{i=1}^{{N}_{y}}{\omega }_{y,i}\times 100 \%$$ (4) where f is the water frequency of the pixel; y is the specified year; Ny is the number of total Landsat observations of the pixel in that year; and \({\omega }_{y,i}\) denotes whether one observation of the pixel is water, with “1” indicating water and “0” indicating non-water. For each image, the cloud, cloud shadow and snow pixels were removed using a cloud masking method called CFmask, which is suitable for preparing Landsat data for surface water area detection 56 , 57 . Terrain shadows were removed using the solar azimuth and zenith angles from Landsat images and the digital elevation model from Shuttle Radar Topography Mission 41 . To reduce the effect of the missing pixels on WF monitoring results, the results for adjacent years were interpolated to obtain a good-quality series of continuous SWA monitoring data. The adjacent-years interpolation method is shown in the appendix 54 . Different thresholds are set to determine the area of different surface water bodies. A recent study found that permanent water body area can be identified as such when the frequency of a water body is greater than 68% 54 . Permanent water body area here corresponds to water body area (WA) in our potential drivers. Improved hydrological connectivity index (HCI) The Dendritic Connectivity Index (DCI) developed by Cote et al. 30 has been widely used in studies related to the assessment of functional hydrological connectivity 14 , 15 . The DCI, which is mainly based on the probability of an organism being able to move freely between two random points in the river network, is calculated as follows: $${\rm{DCI}}=\mathop{\sum }\limits_{i=1}^{n}\mathop{\sum }\limits_{j=1}^{n}{c}_{{ij}}\frac{{l}_{i}}{L}\frac{{l}_{j}}{L}$$ (5) where li and lj denote the lengths of river segments i and j, L indicates the total length of the river segment for the entire region, and cij designates the passability between the river segments i and j. An earlier study by Borselli et al. 58 examined water and sediment connectivity at the raster scale from a geomorphologic perspective, with corresponding improvements later made by Cavalli et al. 59 . The process of calculating the possibility of the ith cell (ci) is as follows: $${c}_{i}={p}^{u}{p}^{d}$$ (6) where \({p}_{m}^{u}\) and \({p}_{m}^{d}\) are the upstream and downstream passabilities of the mth barrier, respectively. $${p}^{u}=\overline{W}\,\bar{S}\sqrt{A}$$ (7) (8) Hydrological connectivity strongly depends on the interactions between multiple components of hydrological characteristics (e.g., volume, area, frequency, duration, rate of change, etc.) and basin geomorphology (e.g., channel and floodplain shape, size, gradient, sediment composition, location along the network, etc.) 20 , 38 . Also, hydrological connectivity is positively correlated with the capacity for water mobility and availability 20 . Surface water body dynamics change to maintain connectivity by creating temporary habitats and providing ephemeral dispersal opportunities 32 . Thus, water body frequency, which identifies the probability of the occurrence of a water body in a region over time from remotely sensed imagery data, can be used to characterize water availability. Replacing the length ratio of the stream with water body frequency enables the water body area and frequency of change to be included in the assessment. At different basin scales, the assessment of hydrological connectivity using observational data can also be realized to improve the model. Thus, the improved HCI can be conceptualized as: $${\rm{HCI}}\propto {c}_{i}$$ (9) (10) $${\rm{HCI}}=f(y)\times {c}_{i}=\frac{1}{{N}_{y}}\mathop{\sum }_{i=1}^{{N}_{y}}{\omega }_{y,i}\times {\log }_{10}\left(\frac{\overline{W}\,\bar{S}\sqrt{A}}{{\sum }_{i}\frac{{d}_{i}}{{W}_{i}{S}_{i}}}\right)$$ (11) where f is the water frequency of the pixel; y is the specified year; Ny is the number of total Landsat observations of the pixel in that year; and ωy,i denotes whether one observation of the pixel is water, with “1” indicating water and “0” indicating non-water. The land cover along the path of the segment is assigned a weight (W) for the actual land use. The topographic slope is also important in this segment, with all transfers stopping at the zero slope in the absence of external forces. Furthermore, \(\bar{W}\) indicates the mean weight factor (dimensionless), \(\bar{S}\) refers to the mean slope (m/m), A represents the area, \({W}_{i}\) is the impedance of the ith cell (dimensionless), \({d}_{i}\) denotes the path distance (m) of the ith cell moving downward, and \({S}_{i}\) is the slope of the ith cell (m/m). The range of HCI is (-∞, + ∞), with larger values representing greater hydrological connectivity. To clearly characterize the changes of hydrological connectivity in the TRB over the last three decades, we first performed a statistical analysis at the grid-point scale (30 × 30 m); while river management units are usually demarcated at the sub-basin scale, we carried out the assessment according to regions. First, we compared the hydrological connectivity of runoff formation area and runoff consumption area based on an elevation of 1500 m. Next, we analyzed the hydrological connectivity of the sub-basin according to the “nine headwaters and one mainstem” framework. Finally, to further investigate the characterization of hydrological connectivity in river reaches, the TRB was divided into 1086 sub-catchments, identified according to the HydroBASINS spatial layer within the HydroSHEDS database ( https://www.hydrosheds.org/ ). Hydrobasin level 8 is the recommended hydrobasin level for sub-catchment-scale environmental impact assessments 18 . The methodological framework used in this study can be found in Supplementary Fig. 5 . Trend and correlation analysis Theil–Sen median trend analysis can be used in combination with the Mann-Kendall test to assess the change features of time-series HCI = {HCI1, HCI2… HCIn} from the perspective of the geographic grid. In the present study, the Theil–Sen median was used to analyze long-term changes in the HCI, and Mann-Kendall was used to conduct tests. For the time series HCI = {HCI1, HCI2… HCIn}, n is the length of the data and the Sen’s slope of the HCI (SHCI) is: $${S}_{{HCI}}=M{edian}\left(\frac{{{HCI}}_{i}-{{HCI}}_{j}}{i-j}\right)$$ (12) where i and j are the time ordinals; \({{HCI}}_{i}\) and \({{HCI}}_{j}\) represent the HCI at time i and j, respectively; SHCI > 0 denotes an upward trend; and SHCI < 0 indicates a downward trend. Identification of HCI drivers based on Random Forest Modeling (RFM) Machine-learning methods have a strong learning ability coupled with flexibility in dealing with different data formats and complex data, without considering the interactions and correlations between independent variables 60 . It assesses the contribution of individual observed variables to the predicted outcomes, i.e., the importance of features, using a decision tree analysis. This can assist in the construction of partial least squares structural equation modeling (PLS-SEM), and the RMF identifies important observed variables that inform the selection of proxy indicators for potential variables in PLS-SEM. Therefore, we employed boosted regression trees and RFM to determine the relative importance of potential drivers to hydrological connectivity. The normalized percent increase in mean squared error (%InMSE) was utilized to evaluate the relative importance of a variable, as the higher the normalized %InMSE, the higher the relative importance of the variable and the stronger the impact of that variable on the prediction. In this paper, RFM was used to rank the importance of potential factors related to climate (PET, TEM, PRE, and SWE), human activity (HFP and LUCC), topography (ELE, Slope, and TWI), vegetation (NDVI), and hydrology (WA) on the HCI. Here, we assume that the topographic factors (ELE, Slope, and TWI) have negligible variations in time scales, while significant heterogeneity exists in space. Therefore, we spatially sampled the HCI and potential driver-related data for 1086 sub-catchments (Hydrobasin level 8) between 2000 to 2020 to identify the dominant factors. Partial least squares structural equation modeling (PLS-SEM) PLS-SEM is an effective method for exploring the interrelationships among multivariate variables. By combining factor analysis and multivariate regression, it allows for simultaneous analysis of multiple dependent and independent variables. PLS-SEM is used to analyze the causal relationship between variables, and it can include both latent and observed variables, to assess their direct and indirect effects on the target variable. PLS-SEM requires a smaller sample size and less normality and independence of the data, while also allowing for causality prediction using latent variables. The function is to reduce the dimensionality of the original data, extract the principal component data into the model, and then obtain the weights of the principal components to realize optimal model construction. To quantify the complex pathways of hydrological connectivity-hydrology feature-climate-human activity-vegetation-topography feature interactions and account for the driving mechanisms of hydrological connectivity, we constructed a model (Fig. 7 ) based on the HCI of the TRB from 2000 to 2020. All natural and anthropogenic factors were assumed to cause changes in hydrological connectivity. Fig. 7: Conceptual partial least squares structural equation modeling of the HCI in the TRB from 2000 to 2020. The arrows between the latent variables and those between the latent variables and the HCI indicate causal relationships, while the arrows between the latent variables and the observed variables indicate their correlations.
Kriya Frequently Asked Questions (FAQ)
When was Kriya founded?
Kriya was founded in 2011.
Where is Kriya's headquarters?
Kriya's headquarters is located at 48-50 Scrutton Street, London.
What is Kriya's latest funding round?
Kriya's latest funding round is Line of Credit - III.
How much did Kriya raise?
Kriya raised a total of $799.45M.
Who are the investors of Kriya?
Investors of Kriya include Viola Group, Viola Credit, Northzone, Barclays Bank, Mouro Capital and 14 more.
Who are Kriya's competitors?
Competitors of Kriya include Fundbox, Instant Factoring, First Circle, iwoca, Prospa and 7 more.
Loading...
Compare Kriya to Competitors
Bluevine is a financial technology company that specializes in providing business banking solutions. The company offers business checking accounts with high-yield interest, accounts payable automation, and extensive FDIC insurance, as well as business loans and credit cards designed to meet the needs of small businesses. Bluevine primarily serves the small business sector with its suite of financial products. It was founded in 2013 and is based in Jersey City, New Jersey.
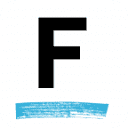
Fundbox provides an embedded working capital platform for small businesses, operating within the financial services industry. It offers services such as business loans and lines of credit, which are designed to help businesses manage their cash flow and cover expenses. It primarily serves the small business sector. The company was founded in 2013 and is based in San Francisco, California.
Funding Societies operates as a digital crowdfunding and financing platform for micro, small, and medium enterprises (MSMEs). It offers MSMEs access to capital without the need to put up collateral. The company also offers solutions besides lending, such as payments and collections, to help SMEs solve their cash flow management challenges. It was founded in 2015 and is based in Singapore.
axio specializes in consumer finance, offering a range of financial services including pay later options, credit facilities, and personal finance management. The company provides these services to individuals, focusing on accessibility and innovation to reach traditionally under-served consumer segments. axio operates as a Non-Banking Financial Company (NBFC) registered with the Reserve Bank of India, leveraging technology to streamline the financial product experience for its customers. It was founded in 2013 and is based in Bengaluru, India.
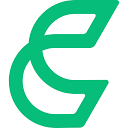
C2FO focuses on providing working capital solutions in the financial sector. The company offers services that allow businesses to get their invoices paid early, providing fast and flexible access to low-cost capital. It is achieved through their patented name-your-rate technology, which eliminates the need for loans, paperwork, or other hassles, allowing businesses to control their cash flow and unlock potential in their balance sheets. C2FO was formerly known as Pollenware. It was founded in 2008 and is based in Leawood, Kansas.
First Circle is a fintech company specializing in providing non-collateral business loans to Small and Medium Enterprises (SMEs). The company offers a revolving credit line that businesses can access anytime for working capital needs, alongside other financial products designed to support business growth and project acquisition. First Circle primarily serves SMEs looking for accessible financing solutions to manage cash flow and fund expansion. It was founded in 2016 and is based in Taguig City, Philippines.
Loading...