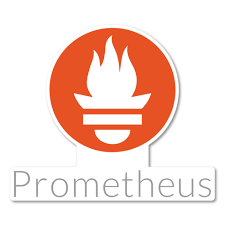
Prometheus
Founded Year
2012About Prometheus
Prometheus is a company that operates in the technology sector, focusing on monitoring systems and time series databases. The company offers an open-source monitoring solution that includes a dimensional data model, a flexible query language, an efficient time series database, and a modern alerting approach. This solution is primarily used by businesses in the cloud computing industry. It was founded in 2012 and is based in California, United States.
Loading...
Loading...
Latest Prometheus News
Oct 22, 2024
Journal of Medical Internet Research This paper is in the following e-collection/theme issue: June 16, 2023 . True Mitotic Count Prediction in Gastrointestinal Stromal Tumors: Bayesian Network Model and PROMETheus (Preoperative Mitosis Estimator Tool) Application Development True Mitotic Count Prediction in Gastrointestinal Stromal Tumors: Bayesian Network Model and PROMETheus (Preoperative Mitosis Estimator Tool) Application Development Authors of this article: 2Pathology Department, Istituto di Ricovero e Cura a Carattere Scientifico (IRCCS) Humanitas Research Hospital, Rozzano, Milan, Italy 3Sarcoma, Melanoma and Rare Tumors Surgery Unit, Istituto di Ricovero e Cura a Carattere Scientifico (IRCCS) Humanitas Research Hospital, Rozzano, Milan, Italy *these authors contributed equally Pieve Emanuele, Milan, 20072 Abstract Background: Gastrointestinal stromal tumors (GISTs) present a complex clinical landscape, where precise preoperative risk assessment plays a pivotal role in guiding therapeutic decisions. Conventional methods for evaluating mitotic count, such as biopsy-based assessments, encounter challenges stemming from tumor heterogeneity and sampling biases, thereby underscoring the urgent need for innovative approaches to enhance prognostic accuracy. Objective: The primary objective of this study was to develop a robust and reliable computational tool, PROMETheus (Preoperative Mitosis Estimator Tool), aimed at refining patient stratification through the precise estimation of mitotic count in GISTs. Methods: Using advanced Bayesian network methodologies, we constructed a directed acyclic graph (DAG) integrating pertinent clinicopathological variables essential for accurate mitotic count prediction on the surgical specimen. Key parameters identified and incorporated into the model encompassed tumor size, location, mitotic count from biopsy specimens, surface area evaluated during biopsy, and tumor response to therapy, when applicable. Rigorous testing procedures, including prior predictive simulations, validation utilizing synthetic data sets were employed. Finally, the model was trained on a comprehensive cohort of real-world GIST cases (n=80), drawn from the repository of the Istituto di Ricovero e Cura a Carattere Scientifico (IRCCS) Humanitas Research Hospital, with a total of 160 cases analyzed. Results: Our computational model exhibited excellent diagnostic performance on synthetic data. Different model architecture were selected based on lower deviance and robust out-of-sample predictive capabilities. Posterior predictive checks (retrodiction) further corroborated the model’s accuracy. Subsequently, PROMETheus was developed. This is an intuitive tool that dynamically computes predicted mitotic count and risk assessment on surgical specimens based on tumor-specific attributes, including size, location, surface area, and biopsy-derived mitotic count, using posterior probabilities derived from the model. Conclusions: The deployment of PROMETheus represents a potential advancement in preoperative risk stratification for GISTs, offering clinicians a precise and reliable means to anticipate mitotic counts on surgical specimens and a solid base to stratify patients for clinical studies. By facilitating tailored therapeutic strategies, this innovative tool is poised to revolutionize clinical decision-making paradigms, ultimately translating into improved patient outcomes and enhanced prognostic precision in the management of GISTs. J Med Internet Res 2024;26:e50023 Introduction Gastrointestinal stromal tumor (GIST) is the most common sarcoma type [ 1 ]. The majority harbors activating mutation in KIT or PDGFRA [ 2 - 5 ]. Even if these mutations represent early events in carcinogenesis—being shared by clinically irrelevant and very aggressive GIST [ 6 , 7 ]—they are the molecular basis for the very active tyrosine-kinase-inhibitor (TKI) therapy [ 8 - 10 ]. TKIs have revolutionized GIST treatment and have been used in all the disease stages since their introduction [ 11 - 14 ]. Notably, they can be administered as neoadjuvant treatment for patients with high-risk disease or for reducing the extent of surgery in GIST in a peculiar location (ie, rectum and duodenum) [ 14 - 17 ]. While neoadjuvant therapy with Imatinib is beneficial for patients with high-risk disease [ 18 ] and for those who may not achieve R0 resection or can undergo less mutilating, function-sparing surgery if there is a volumetric reduction [ 17 ], TKIs present certain challenges. One major issue is that they may impair accurate postoperative risk assessment, as tumor response to therapy can prevent correct risk evaluation [ 5 ]. Several risk assessments in GIST have been developed to identify size, site, and mitotic count as important features [ 19 - 21 ]. Indeed, mitotic count on the surgical specimen after TKI therapy can be greatly modified—especially in the case of tumor response. Therefore, in these patients, the subsequent management will be guided by a risk assessment computed with the mitotic count from the biopsy, and this can lead to mistreatment. On the other hand, during initial patient management, the correct identification of high-risk patients can also fail: a very small amount of tissue on biopsy is required to make the GIST diagnosis since good immunohistochemical markers (rather specific and sensitive) exist [ 22 - 25 ]. Therefore, whereas size and site can be accurately assessed by imaging, mitotic count on biopsy can face several limitations, some purely biological (such as tumor heterogeneity) and others more physical (ie, the size of the specimen available for counting, which is a classic example of sampling bias) [ 26 - 30 ]. Thus, it may happen to incorrectly classify the risk of a GIST preoperatively, and this might lead to surprises after the mitotic count on the surgical specimen is performed, often due to an underestimation of the mitotic count [ 31 - 33 ]. These limitations underscore the critical need for innovative approaches to refine preoperative risk stratification in GIST, aiming to mitigate the risks of misclassification and subsequent therapeutic mismanagement. The discrepancies between preoperative risk assessments and postoperative findings underscore the imperative for precision tools that can dynamically estimate mitotic count on surgical specimens, enhancing the accuracy of patient stratification and treatment planning. In line with this imperative, we aim to develop an advanced computational tool, termed PROMETheus (Preoperative Mitosis Estimator Tool), designed to predict mitotic count on surgical specimens. By leveraging state-of-the-art Bayesian modeling techniques and integrating comprehensive clinicopathological variables, PROMETheus seeks to address the limitations of current risk assessment methodologies, offering clinicians a reliable means to anticipate postoperative mitotic counts and refine preoperative treatment strategies effectively. Methods Bayesian Network and Workflow As a modeling strategy, we used the Bayesian network with the aim of predicting the mitotic count on the surgical specimen. Of note, we use the term “Bayesian network” to indicate the model’s graphical representation and the collection of functions necessary to use it for statistical learning. This loose definition is often used in practice; however, it is broader than the one defined by the term’s inventor, Judea Pearl. In his 2009 book Causality, he identifies the directed acyclic graphs (DAGs) with the term Bayesian networks: “Directed graphs, especially DAGs, have been used to represent causal or temporal relationships…and came to be known as Bayesian networks, a term coined…to emphasize three aspects: (1) the subjective nature of the input information; (2) the reliance on Bayes’ conditioning as the basis for updating information; and (3) the distinction between causal and evidential modes of reasoning [ 34 ]. In this paper, the meaning of “Bayesian network” is closer to the one of structural causal models (SCMs) [ 35 ]. Briefly, we designed an SCM of the variables, created a mock data set, wrote a probabilistic program, validated it on the data simulation, fit the model to the data, and compared multiple models with different structures; these procedures are often collectively referred as the Bayesian workflow [ 36 - 39 ]. Causal Modeling The graphical representation of the Bayesian network was done with DAGs: the variables were represented by nodes and the conditional dependencies through directed edges. Based on the graphical representation, we built an SCM, which we used for data simulation and as the model for the fits. Probabilistic Programming We wrote and fit the models using R software (version 4.1.2; R Foundation for Statistical Computing) and Stan (version 2.21.0; Stan Development Team) [ 40 - 43 ]. Stan is a probabilistic programming language that runs a No U-Turn sampler, an extension to Hamiltonian Monte Carlo (HMC) sampling, which is itself a form of Markov Chain Monte Carlo (MCMC) [ 44 ]. To promote regularization and reduce overfitting, we used a multilevel-hierarchical modeling strategy [ 39 , 45 , 46 ]. Distributions (likelihood and priors) were chosen with maximum entropy criteria [ 39 ]. To understand priors’ implications, we run prior predictive simulations [ 37 , 38 , 46 ]. To minimize divergent transitions, we reparametrized the models with a noncentered equivalent form when appropriated [ 39 , 47 ]. To ensure a good representation of the sample space, we visually inspected the chains with trace plots and trankplots [ 48 ]. We then monitored the chains with postmodeling diagnostics such as the number of effective samples and the Gelman-Rubin convergence diagnostic . . is defined in the cited references is different from the classic definition by Gelman and Rubin (1992). All models’ fits were plotted against the fitted data to ensure a good representation of the outcome space (posterior predictive check) [ 39 , 49 , 50 ]. Compatibility intervals (CIs) were calculated as the highest posterior density interval (HDPI) [ 51 ]. Model Selection For the aim of pure prediction—as in our case—the best model can be selected based on information theory by estimating model performance using the widely applicable information criterion (WAIC, a generalization of Akaike information criteria) and Pareto Smooth Important Sampling Leave-One-Out Cross-Validation (PSIS-LOO-CV) criteria [ 52 - 54 ]. We checked that the 2 statistics gave the same results, leading us to trust their results [ 39 , 55 , 56 ]. We then selected the model with the lowest deviance in out-of-sample performance. Forecasting The posterior probability density of the coefficients was then used to create an application that, given the chosen variables, computed the posterior probability distribution in the outcome space (ie, the mitotic count on the specimen). Moreover, we programmed the application to calculate the risk class from this computed posterior distribution of the mitotic count. Study Population Data Set The cases came from a prospectively maintained database including all the patients who underwent surgery for primary sarcoma in the Istituto di Ricovero e Cura a Carattere Scientifico (IRCCS) Humanitas Research Hospital (Milan, Italy). This database comprises extended clinical and pathological information and contains 233 GISTs operated from January 2000 to March 2022. Inclusion and Exclusion Criteria We included patients who had preoperative diagnostic biopsy and underwent surgical resection, with informed consent for research and available histologic material from both the biopsy and the surgical specimen. The histology was reviewed by a sarcoma pathologist (author SLR), and cases with diagnosis other than GIST were excluded. Pathology Microscope Calibration We calibrated the microscope with a stage micrometer slide and calculated the number of high-power fields (HPFs) needed to reach the size of 5 mm2. In line with the published guidelines, the number of HPFs to evaluate was 23.5 [ 30 , 57 - 59 ]. Mitosis We defined mitosis as basophilic, dark, hairy material representing the chromosomes. Mitosis was counted when the chromosomes were either clotted (as at the beginning of metaphase), in a plane (as in metaphase and anaphase), or in separate clots (as in telophase), as previously described [ 60 ]. Biopsy Measurement We measured the surface available under the microscope counting HPFs filled by neoplastic specimens up to 5 mm2 for very small biopsies we approximated the surface as a fraction of a field of view. Tumor Response Some of the series cases underwent preoperative therapy. To use these cases without polluting the estimate for mitotic count coefficient, we also recorded the response to treatment; this had a different meaning from a classical pathological response and was defined as follows: if a mitotically active area was identified on the surgical specimen (regardless of the size) and the mitotic count in this area was equal to or more than the biopsy count, the tumor was classified as “no response.” Therefore, this mitotic count on the biopsy was used in the model as if the case did not undergo preoperative therapy. Conversely, if the count on the surgical specimen was less than the count on the biopsy, the tumor was classified as “response” and the count was used to estimate a different coefficient that we did not use for prediction (see model description in the Results section and custom code for greater detail). Ethical Considerations All patients signed an institutional written informed consent to research. The Independent Ethics Committee of IRCCS Humanitas Clinical Research reviewed the following project, giving approval on February 21, 2023 (145/23). Data were deidentified before analysis. Results Causal Modeling We designed a causal model to identify the covariates to be included in the model for estimating the mitotic count on the surgical specimen. We assumed that the true mitotic count is the one counted on the surgical specimen (MS) The more tumor cell replicates, the bigger the tumor is (D). We also identified the anatomical location (L) as a cause of tumor dimension (D), in the sense that in certain locations the symptoms would appear earlier, thus influencing the measured size at diagnosis. Moreover, location directly causes the total amount of tissue available for evaluation at biopsy (ie, the measured biopsy surface, S); some sites are more difficult to reach than others. Finally, mitotic count on the biopsy (MB) reflects the mitotic count on the surgical specimen (MS) and due to tumor heterogeneity and sampling bias, the measurement on the biopsy also depends on the surface examined (S; Figure 1 and Multimedia Appendix 1 for an extended version). Figure 1. To determine the model covariates, we utilized a directed acyclic graph (DAG). In this causal framework, several factors influence the mitotic count on the surgical specimen. First, the dimension of the tumor impacts both the mitotic count on the biopsy and the surgical specimen. Larger tumors tend to exhibit higher mitotic activity. Second, the location of the tumor plays a crucial role in its growth pattern. For instance, gastric neoplasms often have more space to expand, leading to the development of symptoms with larger masses. Additionally, tumor location influences the accessibility of the biopsy site, as some sites are inherently more accessible than others. Finally, the amount of surface area available on the biopsy directly impacts the accuracy of the mitotic count estimation. A larger surface area allows for a more representative sampling of the tumor. This simplified causal model elucidates the relationship between various factors affecting GIST mitotic count. Please refer to the main text and supplementary materials for further details. In the model notation,Drepresents the dimension of the tumor, L denotes the location, MB signifies the mitotic count on the biopsy, MS indicates the mitotic count on the surgical specimen, and S represents the surface area of the biopsy. Probabilistic Modeling Our inferential target was the mitotic count on the surgical specimen (MS); as the name suggests, it is a count variable; therefore, we chose a Poisson distribution to model it, as in Equation (1). Poisson distributions have just 1 parameter, λ. It is the expected value and the expected variance of the count variable, and it is the parameter used for the generalized linear model. It needs to be positive, and a common link function is to exponentiate the model. Given each patient i, we estimated a coefficient for the tumor dimension (β) and for surface of the biopsy (γ) for each location (L) using a multilevel-hierarchical model for both of them (β[L]Di and γ[L]Si respectively); the parameters δ and were alternatively switched on and off by the presence of response to therapy R, as defined in the methods section; finally, we set an intercept a, as in Equation (2). To justify the prior choice, we used prior predictive simulation. Prior Predictive Simulation The majority of GISTs are clinically irrelevant, with a mitotic count less than 5/5 mm2. A few of them can have a higher mitotic count, even to a greater order of magnitude; however, it is biologically implausible to expect many cases with a mitotic count greater than 50. Using this field-specific knowledge, we chose normal distributions for model coefficients, as seen in Equations (3), (5), (4), (7), (8), (10), and (11), and exponential distributions for scalar coefficients, as seen in Equations (6) and (9). Through serial simulations, we narrowed the numerical values. For a graphical representation of part of the coefficients, see Multimedia Appendix 2 ). The results of the prior predictive simulation show that most of the simulated λ have values that conform to the field-specific knowledge ( Figure 2 ). Figure 2. Prior predictive simulation of the λ parameter (ie, what the model anticipates before encountering the data). This plot displays 80 simulated cases derived from the priors. The majority of the expected values indicate a very low mitotic count, aligning with real-world expectations. However, the model is not startled by higher mitotic counts, even though it anticipates encountering them in a minority of cases without prior training. Fitting the Mock Data Set To test the model performance, we fitted it on a simulated data set of 100 cases. The custom code to produce the mock data set is available on the cited repository. The fit with a centered parameterization resulted in 2% divergent transitions; therefore, we rewrote the model in a noncentered form. The fit diagnostics were satisfactory: . was obtained for all the parameters, and the energy from the Hamiltonian had a Gaussian outlook and the trankplots of the log-probability showed a satisfactory convergence of the chains ( Multimedia Appendix 3 ). All the parameters had a satisfactory number of effective samples and a good outlook of the trankplots ( Multimedia Appendix 4 ). The posterior probability density for each coefficient is depicted in Multimedia Appendix 5 . To check the model fitness, we compared the inferred λ by the model to the true λ used for data simulation. This procedure revealed that the model regularized the values within each modeled site and was able to recover a value closer to λ even if provided with lower values ( Figure 3 ). Figure 3. Posterior predictive simulation. The upper panel shows the imputed λ parameter for each sample against the ground truth; consider the logarithmic scale on the y-axis. The lower panel shows the same imputed λ parameter against the fitted data. On average, the mitotic count on either the surgical specimen or the biopsy is lower than the expected value. This is due to the asymmetry of the Poisson distribution. The size of the X is proportional to the biopsy surface, and the size of the empty dots is proportional to the tumor size. The color of the background corresponds to the tumor site. NAC: N-acetylcysteine. Fitting the Real Data Set We then fitted the model to the real data. We were able to fit the data with the centered version of the model. Similarly to the simulated dataset, the fit’s diagnostics were satisfactory: . was obtained for all the parameters. The energy from the Hamiltonian had a Gaussian outlook and the trankplots of the log-probability showed a satisfactory convergence of the chains ( Multimedia Appendix 6 ). Moreover, all the parameters had a satisfactory number of effective samples and a good outlook of the trankplots ( Multimedia Appendix 7 ); the posterior probability density for the model coefficient is shown in Multimedia Appendix 8 and Table 1 . The site (L) was an index variable with values from 1 to 4, representing coefficients for the colon-rectum, duodenum, small intestine, and stomach, respectively. Of note, the model posterior distribution for the biopsy count parameter (δ) is 1, consistent with our expectations. As in the data simulation, to see what the model learned, we moved to form the parameter space to the outcome space and simulated from the whole posterior distribution a λ parameter—that tells us the expected value of the Poisson distribution—for each case and plotted it against the data fitted. This posterior predictive simulation is depicted in Figure 4 . Table 1. Coefficients. Figure 4. Posterior predictive simulation on the true data set. The size of the X is proportional to the biopsy surface, the size of the empty dots is proportional to the tumor size; the color of the background corresponds to the tumor site. Model Selection In general, adding parameters in the multilevel modeling reduces overfitting and improves the out-of-sample performance, whereas adding parameters without a hierarchical structure can reduce deviance within the sample, but results in lower out-of-sample performance (ie, increases overfitting). We therefore computed the deviance using WAIC and PSIS-LOO-CVC on our model (which had a multilevel hierarchical structure for the tumor size (βL) and biopsy surface (γL) parameters. We also evaluated alternative models: one incorporating mitotic count on the biopsy with a hierarchical structure, found in Equation 12 in Multimedia Appendix 9 ; one with only tumor size with a hierarchical structure, as in Equation 13 in Multimedia Appendix 9 ; one with only tumor size without accounting for biopsy surface, as in Equation (14) in Multimedia Appendix 9 ; and one without a hierarchical structure without tumor size, as in Equation (15) in Multimedia Appendix 9 . The model with a hierarchical structure for tumor size and biopsy surface parameters, as in Equation (2), had the lowest out-of-sample deviance ( Figure 5 ). Therefore, we chose the model with a hierarchical structure for the tumor size and biopsy surface parameters to develop the application. Figure 5. Model selection. The vertical line indicates the mean deviance of the reference model (the one with the lowest out of sample deviance). The filled dots are values within the sample. The empty dots represent the mean out-of-sample deviance with the bar indicating the 89% CI; the triangle is the contrast between the model and the reference model. Application Development Using the posterior probabilities from the model, we developed PROMETheus, a web-based application [ 61 ]. The user interface has an input panel to insert the tumor location, tumor size, the mitotic count on the biopsy, and the available surface on the biopsy. Then, the application dynamically computes the risk class according to Miettinen and Lasota. Moreover, using the inputted data and the full posterior distribution, the application computes the expected mitotic count on the surgical specimen (indicating the most probable rendered counts) and the predicted risk class for the new posterior distribution of MS provided ( Figure 6 ). Figure 6. The PROMETheus (Preoperative Mitosis Estimator Tool) app. The image shows a screenshot for a gastrointestinal stromal tumor (GIST) measuring 72 mm with a biopsy surface of 14.3 high-power fields (HPFs; about 3 mm2). With these biological characteristics, the risk class is low. However, the model shows that the mitotic count is likely to be underestimated, with the most probable count on the surgical specimen predicted to be between 5 to 8 mitoses in 5 mm^2. Given this predicted mitotic count distribution, it is much more probable for the risk class to be high (more than 60%). Development of the Preoperative Classification for GIST To facilitate stage-adapted treatment planning and enhance data comparison across institutions, we developed a preoperative classification system for GIST based on the results of our computational model, PROMETheus. This classification system aims to provide a standardized definition of preoperative classification for GISTs, which can be used to guide therapeutic decisions and improve clinical trial designs ( Table 2 ). The development of this classification included the probabilistic output of PROMETheus risk classification, important surgical parameters (such as site and size), and respectability. Table 2. Proposed preoperative classification for GISTa. Clinical type
Prometheus Frequently Asked Questions (FAQ)
When was Prometheus founded?
Prometheus was founded in 2012.
Who are Prometheus's competitors?
Competitors of Prometheus include Timeseer, Baselime, SigNoz, Coralogix, Timescale and 7 more.
Loading...
Compare Prometheus to Competitors

Grafana Labs specializes in open observability platforms for monitoring and visualizing operational data across various industries. The company offers solutions for log aggregation, data visualization, distributed tracing, and metrics backend, enabling users to monitor and analyze their operational performance. Grafana Labs provides both fully managed cloud services and self-managed enterprise solutions to cater to different customer needs. Grafana Labs was formerly known as Raintank. It was founded in 2014 and is based in New York, New York.
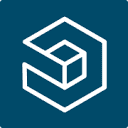
Logz focuses on cloud observability and security, leveraging open-source tools within the technology sector. The company offers a suite of services including log analytics, metrics monitoring, distributed tracing, and cloud-based security information and event management (SIEM). It was founded in 2014 and is based in Boston, Massachusetts.
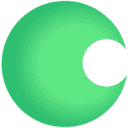
Chronosphere is a cloud native observability platform that specializes in helping DevOps teams manage the speed, scale, and complexity of their cloud native environments. The company offers solutions for incident resolution and cost control, with a focus on resolving infrastructure and application issues before they impact customer experiences. Chronosphere provides a suite of tools for telemetry data collection, transformation, and routing, as well as scalable data storage, all designed to offer real-time insights and enhance application performance while managing observability costs. It was founded in 2019 and is based in New York, New York.
InfluxData focuses on time series data management in the technology sector. The company offers a platform, InfluxDB, that allows users to collect, store, and analyze time series data at any scale, providing real-time insights. The platform is used across various industries such as manufacturing, telecommunications, energy, and utilities. InfluxData was formerly known as Errplane. It was founded in 2012 and is based in San Francisco, California.
AIMS Innovation offers an AI-powered observability platform within the AIOps solutions sector. Their main service includes real-time anomaly detection and analysis of time series data for IT operations. Eyer integrates with existing technology landscapes through open APIs, enabling performance data ingestion and providing actionable insights to improve system efficiency and availability. It was founded in 2011 and is based in Oslo, Norway.
observIQ specializes in telemetry and observability within the IT monitoring sector. It offers solutions to help reduce observability costs, manage telemetry data from various sources, and engage in the management of agent fleets. observIQ primarily serves sectors that require robust IT monitoring and data management, such as cloud computing and enterprise IT infrastructures. observIQ was formerly known as Blue Medora. It was founded in 2020 and is based in Grand Rapids, Michigan.
Loading...