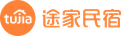
Tujia.com
Founded Year
2011Stage
Series E | AliveTotal Raised
$763.7MValuation
$0000Last Raised
$300M | 7 yrs agoMosaic Score The Mosaic Score is an algorithm that measures the overall financial health and market potential of private companies.
-27 points in the past 30 days
About Tujia.com
Tujia.com offers online vacation rental services. It manages the properties listed on the website and works with real estate developers to rent out unsold inventory including apartments, homestays, and villas. The company was founded in 2011 and is based in Beijing, China.
Loading...
Loading...
Expert Collections containing Tujia.com
Expert Collections are analyst-curated lists that highlight the companies you need to know in the most important technology spaces.
Tujia.com is included in 2 Expert Collections, including Unicorns- Billion Dollar Startups.
Unicorns- Billion Dollar Startups
1,249 items
Travel Technology (Travel Tech)
2,715 items
The travel tech collection includes companies offering tech-enabled services and products for tourists and travel players (hotels, airlines, airports, cruises, etc.). It excludes financial services and micro-mobility solutions.
Latest Tujia.com News
Oct 10, 2024
Ling Hou,1,2,* Ke Su,1,* Ting He,1 Jinbo Zhao,1 Yuanhong Li1 1Cardiovascular Disease Center, Central Hospital of Tujia and Miao Autonomous Prefecture, Hubei University of Medicine, Enshi, Hubei Province, People’s Republic of China; 2Department of Central Hospital of Tujia and Miao Autonomous Prefecture, Hubei University of Medicine, Shiyan, Hubei Province, People’s Republic of China *These authors contributed equally to this work Background: Percutaneous coronary intervention (PCI) is the primary treatment for acute myocardial infarction (AMI). However, in-stent restenosis (ISR) remains a significant limitation to the efficacy of PCI. The cholesterol-to-lymphocyte ratio (CLR), a novel biomarker associated with inflammation and dyslipidemia, may have predictive value for ISR. Deep learning-based models, such as the multilayer perceptron (MLP), can aid in establishing predictive models for ISR using CLR. Methods: A retrospective analysis was conducted on clinical and laboratory data from 1967 patients. The Boruta algorithm was employed to identify key features associated with ISR. An MLP model was developed and divided into training and validation sets. Model performance was evaluated using ROC curves and calibration plots. Results: Patients in the ISR group exhibited significantly higher levels of CLR and low-density lipoprotein (LDL) compared to the non-ISR group. The Boruta algorithm identified 21 important features for subsequent modeling. The MLP model achieved an AUC of 0.95 on the validation set and 0.63 on the test set, indicating good predictive performance. Calibration plots demonstrated good agreement between predicted and observed outcomes. Feature importance analysis revealed that the number of initial stent implants, hemoglobin levels, Gensini score, CLR, and white blood cell count were significant predictors of ISR. Partial dependence plots (PDP) confirmed CLR as a key predictor for ISR. Conclusion: The CLR, as a biomarker that integrates lipid metabolism and inflammation, shows significant potential in predicting coronary ISR. The MLP model, based on deep learning, demonstrated robust predictive capabilities, offering new insights and strategies for clinical decision-making. Keywords: cholesterol-to-lymphocyte ratio, deep learning, multilayer perceptron, Boruta algorithm, in-stent restenosis Background Acute myocardial infarction (AMI) is a severe cardiovascular condition associated with high morbidity and mortality due to complications such as congestive heart failure and malignant arrhythmias. 1 Percutaneous coronary intervention (PCI) is a primary treatment for AMI, 2 yet its efficacy is often hampered by the occurrence of in-stent restenosis (ISR). 3 ISR, characterized by the re-narrowing of the treated vessel, can lead to stent failure, necessitating repeat interventions and adversely affecting patient outcomes. 4 Therefore, identifying strategies to reduce ISR incidence is crucial for improving the prognosis of AMI patients. Previous studies have established that inflammation and dyslipidemia significantly contribute to ISR development. 5–11 Inflammatory responses can cause endothelial injury and proliferation, while abnormal lipid metabolism may accelerate atherosclerosis, promoting ISR. 12 , 13 Traditional ISR prediction methods often rely on single biomarkers, which may lack accuracy and sensitivity. Consequently, there is a need to explore novel indicators and methods for ISR prediction. Blood cholesterol levels reflect lipid metabolism, and lymphocyte ratios indicate immune status. The cholesterol-to-lymphocyte ratio (CLR) is an emerging biomarker that encapsulates both lipid metabolism and inflammation, 14 potentially offering unique advantages in predicting ISR. Other relevant factors such as age, gender, hypertension, diabetes mellitus, and smoking status may play significant roles in ISR risk. Previous research has highlighted that advanced age and male gender are associated with increased ISR rates. 15 Furthermore, hypertension and diabetes are well-known contributors to endothelial dysfunction, atherosclerosis, and subsequent ISR. 16 Smoking is another critical risk factor, as it exacerbates inflammation and accelerates the process of vascular injury. 17 By considering these clinical and demographic factors alongside CLR, this study can provide a more comprehensive model for ISR prediction. Multilayer perceptron (MLP)-based deep learning models are powerful predictive tools capable of handling complex data relationships and non-linear modeling. 18 This study aims to investigate the impact of CLR on ISR in coronary stenting, providing new insights and strategies for clinical decision-making. Methods Study Population This retrospective analysis encompassed 1967 patients from the Cardiology Department at Enshi Tujia and Miao Autonomous Prefecture Central Hospital, who underwent PCI followed by a subsequent coronary angiography between January 2022 and May 2024. Inclusion criteria were: (1) patients who had received coronary stent intervention with a postoperative period exceeding 90 days; (2) patients who underwent coronary angiography; (3) patients who regularly took antiplatelet and lipid-lowering medications. Exclusion criteria included: (1) patients who refused medication due to disease reasons (eg, bleeding, liver function abnormalities) or poor compliance; (2) patients with procedural factors during the initial PCI that could lead to restenosis within 90 days post-procedure; (3) patients excluded due to other diseases that might affect the study outcomes (tumors, embolism, rheumatic immune diseases); (4) patients with incomplete follow-up data (as shown in Figure 1 ). ISR was defined as a luminal diameter stenosis ≥50% within or at the stent edges (≤5 mm from the stent edge) using the distal vessel diameter as a reference. 19 This study, a retrospective observational study, was approved by the Ethics Committee of Enshi Tujia-Miao Autonomous Prefecture Central Hospital and adhered to the principles outlined in the Declaration of Helsinki. Due to the retrospective nature of the study, written informed consent was not required. Patient data confidentiality was strictly maintained, and all data were anonymized prior to analysis to protect patient privacy. No identifying information, such as names, addresses, or personal identifiers, was included in the dataset. Figure 1 Flowchart of the detailed selection process. Data Collection Clinical data were collected for all patients, including age, gender, smoking history, comorbidities (diabetes, hypertension, stroke), number of lesions, number of stents, stent length, stent diameter, and postoperative medication use (anticoagulants, diuretics). Fasting venous blood samples were collected in the morning for complete blood count, liver and kidney function tests, and lipid profile. CLR was calculated by dividing the total cholesterol by the absolute lymphocyte count. 14 Feature Selection We utilized the Boruta algorithm to identify the most critical baseline features associated with ISR. The Boruta algorithm, an extension of the random forest algorithm, determines important features by comparing original features with randomly shuffled “shadow” features to predict the target variable. 20 Deep Learning Model Development The multilayer perceptron (MLP), a foundational model in deep learning, consists of multiple neuron layers interconnected by weights. By stacking multiple neural network layers and incorporating nonlinear activation functions, MLPs can learn complex feature representations, thereby enhancing model accuracy and generalization capabilities. We employed an 8:2 training-validation split to leverage a larger dataset for learning more features and patterns, thereby improving model performance. We plotted ROC curves and calibration curves to compare model performance on training and test sets, analyzing prediction accuracy and reliability. Feature importance plots visually represented the contribution of different features to ISR. Finally, we used Partial Dependence Plots (PDP) to examine the interaction between ISR and various features, revealing the complex relationships and their impact on model predictions. Statistical Analysis Statistical analyses were conducted using R version 4.3.3 and Python version 3.9. Continuous variables with normal distribution were expressed as mean ± standard deviation, while categorical variables were expressed as percentages. A two-tailed p-value of less than 0.05 was considered statistically significant. Results Baseline Characteristics As shown in Table 1 , there was no significant difference in age between the groups, although the mean age of patients in the ISR group was 64 years, slightly higher than the 63 years in the non-ISR group. A significant gender difference was observed, with a higher proportion of males in the ISR group compared to the non-ISR group (83.8% vs 72.1%, P < 0.001). There were no significant differences between the groups regarding baseline comorbidities such as diabetes, hypertension, and stroke. Clinically, the ISR group had a significantly higher proportion of smokers compared to the non-ISR group (P = 0.001). Regarding coronary artery lesion locations, the ISR group had significantly higher rates of lesions in the left anterior descending, left circumflex, and right coronary arteries. Additionally, the ISR group exhibited a higher number of diseased vessels and Gensini scores than the non-ISR group. In laboratory indices, most parameters (including leukocytes, neutrophils, monocytes, hemoglobin, platelets, platelet distribution width, aspartate aminotransferase, direct bilirubin, creatinine, random blood glucose, uric acid, triglycerides, high-density lipoprotein, and thyroid-stimulating hormone) showed no significant differences between the groups. However, the ISR group had significantly higher CLR and LDL levels compared to the non-ISR group. Table 1 Descriptive Characteristics of Overall Participants Feature Selection Using Boruta Algorithm Figure 2 illustrates the feature selection results using the Boruta algorithm. In this algorithm, the green area represents important features that significantly contribute to the model’s predictive performance. The red area indicates unimportant features with no significant contribution, while the yellow area represents tentative features that lie between important and unimportant. For accurate subsequent modeling, we selected only the important features (21 in total) from the green area. Figure 2 Feature selection based on Boruta algorithm (The green area indicates important features that significantly impact the model’s predictions, while the red area shows unimportant features. The yellow area represents features of uncertain importance. The blue area represents the shadow features, which serve as a reference for determining the significance of the actual features). Model Performance Figure 3 presents the ROC curve (Receiver Operating Characteristic curve) and calibration curve for the MLP model. The AUC (Area Under the Curve) for the validation set was 0.95, and for the test set, it was 0.63, indicating satisfactory model performance with good accuracy and sensitivity. Moreover, the calibration curve demonstrated good alignment between predictions and observations in both the training and test sets. Figure 4 displays the contribution of each variable to ISR occurrence, with the top five variables being the number of stents initially implanted, hemoglobin, Gensini score, CLR, and leukocytes. To further explore the relationship between CLR and ISR in the MLP model, we plotted PDP contour maps of CLR with the top four contributing variables: the number of stents initially implanted, hemoglobin, Gensini score, and leukocytes. As depicted in Figure 5 , the contour maps clearly indicate that CLR is a critical variable in predicting ISR. Higher CLR values consistently corresponded to higher ISR probabilities across all variable combinations, highlighting CLR’s significant role in prediction. Regardless of the values of other variables (Brackets, Gensini, Hb, WBC), an increase in CLR was always associated with an increased probability of ISR. Figure 3 ROC curves and calibration curves of the training set and validation set. Figure 4 Feature importance of variables in multilayer perceptron model. Figure 5 The synergistic effect of the main variables on in-stent restenosis. Discussion Our research identified that the CLR plays a significant role in the occurrence of ISR. Using the Boruta algorithm for feature selection, we determined 21 important features for subsequent modeling. The MLP model demonstrated good predictive performance on both the validation and test sets. The model’s calibration curve also showed a good agreement between predicted and actual outcomes. Further analysis revealed that the number of initial stent implants, hemoglobin levels, Gensini score, CLR, and white blood cell count were the five most significant variables contributing to ISR. Notably, contour plots illustrated CLR as a critical predictor of ISR, consistently showing an increased probability of ISR with higher CLR values, regardless of other variables. Stent implantation disrupts the endothelial layer, leading to cholesterol and lipoprotein deposition, plaque formation, and an increased risk of thrombosis. 21 Lipid content within plaques induces inflammatory cell aggregation, releasing inflammatory factors that activate endothelial cells, exacerbating the inflammatory response. 22 Cholesterol deposits within plaques cause apoptosis and necrosis, releasing intracellular substances that further stimulate inflammation. 23 Plaque formation damages and ruptures the vascular wall, exposing tissue factors that promote platelet aggregation and thrombosis, 24 creating a vicious cycle leading to ISR. The cholesterol-to-lymphocyte ratio provides a unique insight into the interplay between lipid metabolism and inflammation, both of which are critical drivers of ISR. Elevated CLR reflects higher cholesterol levels, contributing to lipid deposition and atherogenesis. This triggers an inflammatory cascade that exacerbates endothelial dysfunction and promotes neointimal hyperplasia, the primary pathological mechanism of ISR. Additionally, lymphocytes play a key role in modulating the immune response within the vascular system. Activated lymphocytes can increase local inflammation, leading to smooth muscle proliferation and plaque instability. This suggests that CLR, by capturing both lipid abnormalities and immune status, acts as a comprehensive marker of ISR risk. Lymphocytes, as key cells in the inflammatory response, play a critical role in arterial wall inflammation. 25 Their activation and proliferation can exacerbate local inflammation, promote smooth muscle cell proliferation and migration, leading to neointimal hyperplasia, 26 a primary pathological mechanism of ISR. Therefore, high CLR levels may reflect enhanced inflammatory response, indicating a high risk of ISR. Elevated total cholesterol levels are a significant risk factor for atherosclerosis, as its deposition in the arterial wall leads to plaque formation and progression. 27 Although PCI can mechanically resolve coronary artery stenosis, high cholesterol levels may promote plaque regeneration and neointimal hyperplasia at the stent site, 28 increasing ISR incidence. CLR includes total cholesterol levels, thus indirectly reflecting the impact of lipid metabolism on ISR. Compared to single inflammatory markers (NLR, SII, SIRI, AISI) or lipid indicators (RC, TC/HDL-C, LDL-C/HDL-C, TG/HDL-C), CLR combines inflammation and lipid metabolism aspects, providing a more comprehensive risk assessment. High CLR values may indicate more severe systemic inflammation and lipid abnormalities, jointly increasing ISR risk. The activation of pro-inflammatory cytokines can mediate endothelial damage, further impairing vascular healing following PCI. High levels of cholesterol exacerbate oxidative stress and lipid peroxidation within the arterial wall, fostering a pro-thrombotic environment. This, in turn, promotes smooth muscle cell migration and proliferation, driving restenosis. Therefore, the dual contribution of high cholesterol and immune activation, as reflected by CLR, underscores its relevance in predicting ISR. CLR, by encompassing these key biological pathways, is not only a marker but potentially a contributor to ISR pathophysiology. Previous studies have primarily used CLR to evaluate adverse prognosis in cancer patients post-surgery. 14 , 29 Our study is the first to describe the association between CLR and cardiovascular events. In clinical practice, CLR can serve as a simple and accessible indicator, helping healthcare providers more accurately predict ISR risk. Regular monitoring of CLR levels in patients can facilitate early identification of high-risk individuals and the implementation of more aggressive preventive measures, such as intensified anti-inflammatory and lipid-lowering treatments, optimizing post-PCI management strategies to improve long-term outcomes. Compared to our previous study, 30 this research utilizes data collected from a different time period and excludes data from patients with repeat hospital admissions. We employed the Boruta algorithm for feature selection and integrated it with a deep learning model to enhance the precision of predicting post-PCI in-stent restenosis. Additionally, this study places a specific emphasis on the roles of blood cholesterol and lymphocyte ratios in the prediction model, aspects that were not extensively explored in our prior work. These innovations highlight the robustness and applicability of our model in clinical settings, providing a more precise and comprehensive tool for predicting ISR risk. However, the observed discrepancy in AUC scores between the validation (0.95) and test sets (0.63) may stem from overfitting, where the model performs exceptionally well on the training data but struggles to generalize to new data. Differences in patient characteristics between the training and test sets, along with the smaller size of the test set, could also contribute to this variance. Addressing these issues in future work will be crucial for improving model robustness and predictive accuracy. Conclusion As a novel biomarker reflecting both inflammation and lipid metabolism, CLR shows significant potential in predicting in-stent restenosis. Further large-scale clinical studies are warranted to validate the predictive value of CLR and explore its application prospects in cardiovascular disease management. Disclosure
Tujia.com Frequently Asked Questions (FAQ)
When was Tujia.com founded?
Tujia.com was founded in 2011.
Where is Tujia.com's headquarters?
Tujia.com's headquarters is located at Floor 5 Building 148 , Beijing.
What is Tujia.com's latest funding round?
Tujia.com's latest funding round is Series E.
How much did Tujia.com raise?
Tujia.com raised a total of $763.7M.
Who are the investors of Tujia.com?
Investors of Tujia.com include Ctrip, All-Stars Investment, China Renaissance, Glade Brook Capital, G Street Capital and 10 more.
Who are Tujia.com's competitors?
Competitors of Tujia.com include AsiaYo, Gathern, XiaoZhu, Airbnb, Ziroom and 7 more.
Loading...
Compare Tujia.com to Competitors
StayList is a search engine specializing in homestay accommodations within the travel and hospitality industry. The platform aggregates listings from multiple sites, allowing users to efficiently find and compare homestay options worldwide. It provides various filters to refine search results by accommodation type, amenities, and location. It was founded in 2018 and is based in Tokyo, Japan.
TRAIWAN is a hospitality service provider focused on offering lodging options across various popular travel destinations. The company's main services include a platform for booking distinctive homestays, private villas, family-friendly accommodations, camping areas, camper vans, backpacker hostels, and more, with a user-friendly interface and multiple payment options. It primarily serves the tourism and travel industry by facilitating easy and diverse lodging bookings. It is based in Taiwan.
Locals offers a mobile homestay rental platform that enables travelers to book boutique bed and breakfasts. The company offers a management platform that helps landlords in the design, renovation, promotion, reception, and operation of their lodgings. It was founded in 2015 and is based in Beijing, China.
Tripvillas is a company specializing in holiday home rentals, offering a platform for booking vacation rentals, homestays, and villas across various destinations. The company provides a marketplace for travelers to find and reserve family-owned properties, as well as managed community services for a comfortable stay. Tripvillas also caters to property owners and managers by offering a dashboard for listing properties and managing bookings, alongside opportunities for holiday home sales and investments. It was founded in 2010 and is based in Singapore, Singapore.
UpNext is a company specializing in travel technology solutions within the travel and tourism industry. The company offers a platform that leverages micro-segmentation to provide personalized travel experiences, connecting travelers with bespoke opportunities in short term rentals and hotel accommodations. UpNext primarily serves the travel industry, focusing on enhancing the travel experience for individuals. It was founded in 2015 and is based in Pitt Meadows, British Columbia.
OYO provides hospitality services focusing on hotel and home bookings. The company offers a platform for sanitized stays, standardized amenities, and digital management tools for property owners. It primarily serves the hospitality and travel sectors by enabling property owners to list their hotels and homes and providing travelers with accommodations. It was founded in 2012 and is based in Gurugram, India.
Loading...