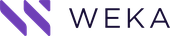
Weka
Founded Year
2013Stage
Series E | AliveTotal Raised
$373.2MValuation
$0000Last Raised
$100M | 6 mos agoMosaic Score The Mosaic Score is an algorithm that measures the overall financial health and market potential of private companies.
+38 points in the past 30 days
About Weka
Weka provides a software-defined, cloud-native data platform for seamless and sustainable data management in the cloud and on-premises environments. The company offers solutions that enable organizations to store, process, and manage data with high input-output performance and low latency, catering to next-generation workloads such as AI and high-performance computing (HPC). Weka was formerly known as WekaIO. It was founded in 2013 and is based in Campbell, California.
Loading...
Loading...
Research containing Weka
Get data-driven expert analysis from the CB Insights Intelligence Unit.
CB Insights Intelligence Analysts have mentioned Weka in 2 CB Insights research briefs, most recently on Jun 20, 2024.
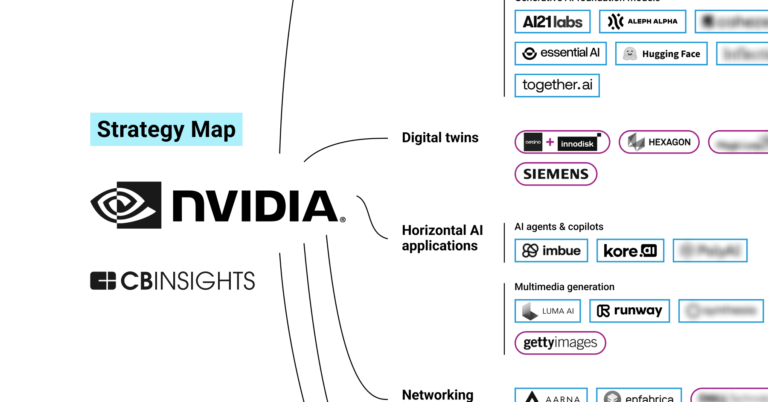
Expert Collections containing Weka
Expert Collections are analyst-curated lists that highlight the companies you need to know in the most important technology spaces.
Weka is included in 3 Expert Collections, including Unicorns- Billion Dollar Startups.
Unicorns- Billion Dollar Startups
1,249 items
Generative AI
940 items
Companies working on generative AI applications and infrastructure.
Artificial Intelligence
6,855 items
Weka Patents
Weka has filed 115 patents.
The 3 most popular patent topics include:
- computer storage devices
- computer memory
- network file systems
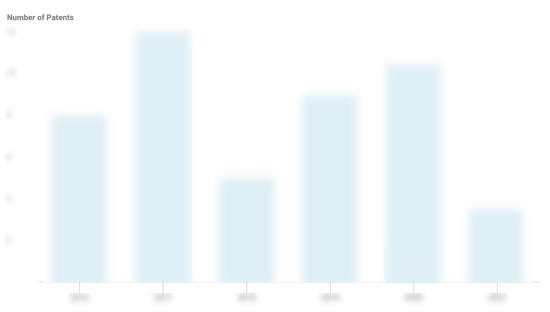
Application Date | Grant Date | Title | Related Topics | Status |
---|---|---|---|---|
5/10/2023 | 9/24/2024 | Computer storage devices, Energy storage, Computer data storage, USB, Data management | Grant |
Application Date | 5/10/2023 |
---|---|
Grant Date | 9/24/2024 |
Title | |
Related Topics | Computer storage devices, Energy storage, Computer data storage, USB, Data management |
Status | Grant |
Latest Weka News
Oct 25, 2024
Abstract Data are becoming more important in education since they allow for the analysis and prediction of future behaviour to improve academic performance and quality at educational institutions. However, academic performance is affected by regions’ conditions, such as demographic, psychographic, socioeconomic and behavioural variables, especially in lagging regions. This paper presents a methodology based on applying nine classification algorithms and Shapley values to identify the variables that influence the performance of the Colombian standardised test: the Saber 11 exam. This study is innovative because, unlike others, it applies to lagging regions and combines the use of EDM and Shapley values to predict students’ academic performance and analyse the influence of each variable on academic performance. The results show that the algorithms with the best accuracy are Extreme Gradient Boosting Machine, Light Gradient Boosting Machine, and Gradient Boosting Machine. According to the Shapley values, the most influential variables are the socioeconomic level index, gender, region, location of the educational institution, and age. For Colombia, the results showed that male students from urban educational institutions over 18 years have the best academic performance. Moreover, there are differences in educational quality among the lagging regions. Students from Nariño have advantages over ones from other departments. The proposed methodology allows for generating public policies better aligned with the reality of lagging regions and achieving equity in access to education. Introduction The volume of available data during the last decade has grown exponentially. It has led companies, governments and individuals to exploit the knowledge provided by it 1 . Different economic sectors realised that they had accumulated data that could be useful with investment in technology. Therefore, researchers have turned data warehouses into sources of information where knowledge can be obtained from data to improve every organisation and make it more competitive 2 . To date, the tool used by institutions is descriptive statistics, i.e., tables, indicators and graphs, because they are easy to develop and interpret 3 . However, descriptive, predictive and prescriptive analytics can be performed with current technology to understand past information and predict future trends. Therefore, every organisation invests resources in robust tools for a better decision-making process 4 . The educational sector has been aware of the knowledge acquisition process based on data warehouses. In 2005, academics on data mining applied these techniques for solving problems in the education field to boost the growth and development of innovative technologies 5 . With this new trend, several studies have been focused on predicting academic performance, school dropout and student behaviour, among others 6 , 7 , 8 , 9 . Research has considered variables such as gender, family income, distance from educational institutions, type of school, absence, classroom environment demographic, psychographic and geographic variables supported by the different government and academic institutions databases 10 . Identifying these variables allows the analysis of the standardised tests that measure the added value given by each institution in the academic development of every student and their accessibility to higher education. Therefore, educational policies can be designed based on the results of descriptive and predictive analysis of those tests to impact students 11 . In Colombia, as in other countries, many institutions have stored data without exploiting knowledge. One of the government entities interested in the value of information is the Colombian Institute for the Evaluation of Education (ICFES). Its mission is to evaluate the training offered by educational institutions at different levels using standardised tests. These tests are applied in various stages of school. The results obtained in these exams determine the student’s skills gained during their training process, and it is an instrument for improving the academic quality of institutions. It links the results with the synthetic index of educational quality (ISCE) to measure the quality improvement in the country’s educational institutions over a determined period 12 . The results of standardised tests on developing countries (i.e., Colombia) have been heterogeneous, particularly in lagged regions. Economic factors, population growth and the aggregation in a geographical area should be analysed to identify them. Maldonado and Meza 13 defined lagged territories based on the economic differences among many geographic areas given by development, i.e., regions are identified, classified and organised according to the benefits each one has received from the country’s development. Regions’ growth also depends on geographic location, transportation network, natural resources and qualified human capital. According to several theories, economic development is related to physical capital, human capital and innovation 14 . Pugh 15 stated that education promotes regional economic growth since it is usually the centre of strategies and the core of economic emergence from knowledge. According to the above, it is clear that the lagging is directly related to the quality of education. This implies that the opportunities for higher education are unequal for people in the same country due to differences in sociodemographic contexts. Therefore, this paper aims to identify the variables that affect academic performance in lagging regions using educational data mining (EDM) jointly with Shapley values (SHAP), as they are backwards in their education system. Moreover, the main contribution of this work is to identify the variables of lagging regions that may hinder the implementation of strategies designed by the government to improve the education system in the medium and long term. As they are lagging regions, the education solutions must be comprehensive, i.e., they must avoid solving isolated problems. Therefore, the factors that hamper access to higher education are addressed through school training. The proposed methodology provides a basis for designing governmental strategies that improve those aspects that influence the student’s performance in their progress through elementary education. In this way, achieving equity in the quality of education in the different regions is possible. The rest of the paper is organised as follows. Section 2 reviews recent related works on educational data mining. Section 3 presents the proposed methodology based on Cross Industry Standard Process for Data Mining (CRISP-DM). Section 4 details the results and discusses the obtained findings. Finally, 5 concludes this paper. Related works EDM is the field focused on applying statistical, machine learning, and data mining algorithms to different types of educational data. This concept appeared in 2005 and resulted from the interest of researchers in areas such as computer science, education, psychology, neuropsychiatry and human behaviour 16 , 17 , 18 , 19 , agriculture 20 , and statistics 21 , 22 . The main objectives of EDM are focused on behaviour detection, feedback for supporting teaching, performance prediction, recommendations to students, class planning, study groups, social network analysis, course construction, creation of early warnings and data visualisation 23 . Romero and Ventura 5 identified trends, the most used algorithms and listed contexts in which EDM could be useful. Baker and Yacef 24 described the evolution of this research field and highlighted recent contributions. Huebner 25 showed how EDM helps to improve students’ success in the learning process. Peña-Ayala 26 identified the influence of education systems and how EDM is used for analysing them. Shahiri et al. 27 and Kumar and Singh 28 developed techniques for predicting students’ performance, progress or regression, and outperformance in the classroom and analysed behavioural patterns and academic dropout. Satyanarayana and Nuckowski 29 used three classification techniques: Decision Trees (DT), Random Forest (RF) and Naive Bayes (NB) to improve data quality and prediction metrics. The accuracy was enhanced by eliminating noise-generating variables and instances. Also, the results provided rules for understanding the factors that influence student outcomes. Venkatachalapathy et al. 30 analysed the most used tools in EDM that focus on visualisation, association, classification, text mining, pattern detection, clustering, web mining and logical rules. Widyahastuti and Tjhin 31 compared the results of linear regression and a multilayer perceptron (MLP) to predict the outcome of the last exam in a bachelor’s degree, using a data set of 50 students. The MLP was the algorithm with the best results. Kumari et al. 32 studied how behavioural characteristics related to the learning experience during students’ training affect academic performance. Four classification algorithms of the WEKA tool were used: ID3, K-nearest neighbour, SVM and NB. They concluded that an accurate prediction is achieved by performing a good analysis of behavioural characteristics. Adejo and Connolly 33 proposed a set of multiple models to predict the academic performance of undergraduate students. They used different data sources and three classification algorithms (DT, SVM and Artificial Neural Networks (ANN)). Results showed that implementing heterogeneous ensemble techniques helps identify students at dropout risk. Ma and Zhou 34 used DTs and SVM to find relevant characteristics that affect students’ approval rates based on two categories (pass and fail). The authors used demographic variables, analysed the dependence between them and included the orientation provided by experts. Optimised using grid search, SVM was the best performance algorithm. Kumar et al. 35 proposed data visualisation in EDM, focusing on graphic language. They implemented Python programming language, the Jupyter Notebooks development IDE, and the MatplotLib and Seaborn libraries. The data set contained 22242 instances with categorical and quantitative variables. Based on the analysis of this set, correct predictions from the visualisation activities were achieved. Hellas et al. 36 and Muzzammel 37 conducted a literature review in data mining, analysing the variables and the different techniques used by researchers from 2009 to 2019. They provided an update on all the methodologies implemented so far. Khan et al. 38 used four algorithms of the WEKA tool: NB, DT, RF and MLP to analyse students’ performance in educational institutions. DT outperformed the other algorithms with an accuracy of 0.88. The study helps students to predict their probably final grades and change their academic behaviour to achieve better results. Livieris et al. 39 implemented semi-supervised learning techniques to predict the student’s academic performance in high school. They used a data set with the grades of 3716 students who studied mathematics between 2007 and 2016. The use of these techniques improved the accuracy, even with unlabeled data. Prasanalakshmi and Farouk 40 also used classification algorithms to predict academic performance. They used the WEKA tool and found that DT and random forest algorithms gave the best results but with an unpromising accuracy of 0.56. 41 used NB, DT and ANN as classification algorithms with academic and demographic variables to develop a Kalboard platform. Results showed that the classifier with the highest value was the neural network, with an accuracy of 0.78. Nahar et al. 42 analysed engineering students’ behaviour and academic performance using DT, NB, PART, bagging, boosting and RF. The students’ results were predicted based on the pre-requisite course performance and the grades until the midterm exam. The best models were DT and NB. Eleyan et al. 43 explored machine learning techniques such as classification trees, regression trees, logistic regression, and multiple regression to predict the final grades of secondary school students. The study concluded that classification trees and logistic regression outperformed the other methods. In addition, they help to identify students at risk and implement tailor-made interventions. Dinh-Thanh and Thi-Ngoc-Diem 44 used RF, XGBoost and Light GBM to predict and identify features that influence the academic performance of high school students. Results indicated that the cumulative GPA, age, class, father/mother occupation, and learning online contribute to the student’s performance. Ghosh 45 applied different classification techniques such as ANN, SVM, RF, and DT to predict academic performance. Findings suggested that these methods provide results that improve administrative and teaching staff in educational institutions. Alamgir et al. 46 implemented linear regression, RF regressor, and MLP to predict the final graduating cumulative GPA of undergraduate students based on the grades in pre-requisite courses and a relative grading scheme. Results showed that RF performed the best among all the classifiers. Alghamdi and Rahman 47 used NB and RF to predict academic achievement and support secondary school students. The models’ performance was validated using several evaluation metrics, and results showed that NB had the best accuracy. Nayak et al. 48 analysed students’ academic success, behaviour, and demographics using DT, NB, RF and MLP algorithms. Results showed that behavioural features influence the model’s accuracy, indicating the importance of students’ attitudes in achieving the desired academic outcomes. Recently, Batool et al. 49 provided a systematic literature review of 260 research studies focused on data mining algorithms, tools, and students’ attributes. Results concluded that DT is the most used algorithm, but ANN, SVM, and RF are trending. In addition, the authors indicated that academic records and demographic factors are the best predictors of students’ future results. The literature reviewed shows a high application of machine learning tools to analyse academic performance in educational institutions like DT, RF, NB and ANN. However, these techniques have yet to be implemented to analyse academic performance in lagging regions where the socioeconomic context has a strong influence. Moreover, other algorithms have been successful in other research problems, such as Extreme Randomised Trees, Extreme Gradient Boosting Machine and Light Gradient Boosting Machine. This article proposes its implementation in the analysis of academic performance and compares it with those traditionally used to identify the best performer. Proposed methodology The proposed methodology was an adaptation of CRISP-DM 50 , which has been applied by different authors in the education field 5 , 11 , 21 , 51 , 52 , 53 . This paper uses CRISP-DM to analyse the results of the standardised test taken by senior students in Colombia: the Saber 11 test. The exam is applied in all regions of Colombia to characterise academic performance, and its structure is similar to the final school year tests carried out in other countries. Its purpose is to measure the essential competencies that guarantee the student has the necessary knowledge to enter higher education. Also, it measures the added value the school has in students’ training. The CRISP-DM methodology is widely used in academia and industry and has several modifications. Figure 1 presents the workflow of the CRISP-DM methodology. Fig. 1
Weka Frequently Asked Questions (FAQ)
When was Weka founded?
Weka was founded in 2013.
Where is Weka's headquarters?
Weka's headquarters is located at 910 East Hamilton Avenue, Campbell.
What is Weka's latest funding round?
Weka's latest funding round is Series E.
How much did Weka raise?
Weka raised a total of $373.2M.
Who are the investors of Weka?
Investors of Weka include Qualcomm Ventures, Hitachi Ventures, MoreTech Ventures, Norwest Venture Partners, Generation Investment Management and 24 more.
Who are Weka's competitors?
Competitors of Weka include SandStone, Unravel, Turntable, ForePaaS, Excelero and 7 more.
Loading...
Compare Weka to Competitors
OSNEXUS is a company that specializes in Software Defined Storage (SDS) within the technology industry. The company's main product, QuantaStor, is a platform that transforms standard servers into multi-protocol scale-out storage appliances, providing unified file, block, and object storage. OSNEXUS primarily serves sectors such as server virtualization, big data, cloud computing, backup/archive, and high performance computing. It is based in Bellevue, Washington.
ProphetStor Data Services is a company that focuses on providing AI-driven data services in the IT infrastructure industry. The company's main offerings include solutions for IT operations, cost management, performance optimization, and application acceleration, all powered by AI and machine learning technologies. These services primarily cater to the cloud computing industry. It is based in Milpitas, California.
SandStone provides enterprise-level software-defined storage (SDS) solutions and services. It offers a range of storage solutions, including massive object storage, unified storage platforms, and cloud-native file storage, designed to help businesses handle the storage challenges associated with IT cloud migration and manage large volumes of unstructured data. It primarily serves sectors such as private and hybrid cloud, fintech, smart government, smart healthcare, smart transportation, and smart security. The company was founded in 2014 and is based in Shenzhen, China.
Atlantis Computing is a company that focuses on software-defined storage (SDS) and operates within the data storage industry. The company offers a flexible and powerful SDS platform that can be delivered as an all-software solution or as a flash-based, hyperconverged appliance. This platform accelerates physical storage performance, increases its capacity, and allows enterprises to transition from costly shared storage systems to lower cost hyperconverged systems and public cloud storage. It was founded in 2006 and is based in Sunnyvale, California.
Simplyblock specializes in storage solutions for cloud-native environments, focusing on the technology sector. The company offers distributed block storage software optimized for Kubernetes, providing elastic and scalable storage with low latency and high IOPS, suitable for IO-intensive and latency-sensitive workloads. Simplyblock's products are designed to serve sectors that require robust data storage solutions, such as the cloud computing industry and businesses leveraging containerized applications. It was founded in 2022 and is based in Teltow, Germany.
DDN Storage is a data storage company that specializes in providing intelligent technology and infrastructure solutions for data-centric organizations. The company offers powerful data management solutions that are designed to support data-centric AI, analytics, and real-time insight. DDN Storage primarily serves enterprises, government, and public-sector customers, including financial services firms, life science organizations, manufacturing and energy companies, research facilities, and web and cloud service providers. It was founded in 1988 and is based in Chatsworth, California.
Loading...